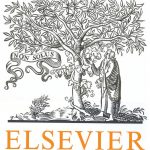
ترجمه مقاله نقش ضروری ارتباطات 6G با چشم انداز صنعت 4.0
- مبلغ: ۸۶,۰۰۰ تومان
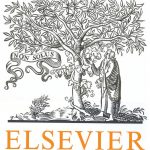
ترجمه مقاله پایداری توسعه شهری، تعدیل ساختار صنعتی و کارایی کاربری زمین
- مبلغ: ۹۱,۰۰۰ تومان
Abstract
This paper provides an empirical evaluation of the U.S. aggregate stock market predictability based on a new technical analysis index that eliminates the idiosyncratic noise component in technical indicators. I find that the new index exhibits statistically and economically significant in-sample and out-of-sample predictive power and outperforms the well-known technical indicators and macroeconomic variables. In addition, it can predict cross-sectional stock portfolio returns sorted by size, value, momentum, and industry and generate substantial utility gains for a mean-variance investor. A vector autoregression-based stock return decomposition shows that the economic source of the predictive power predominantly comes from time variations in future cash flows (i.e., the cash flow channel).
5. Concluding remarks
In this paper, I propose a new aligned technical analysis index (TECHPLS) that is constructed by incorporating 14 well-known technical indicators from Neely et al. (2014) using the PLS method suggested by Kelly and Pruitt (2013, 2015). I document that the TECHPLS index is a statistically and economically significant predictor of the aggregate stock market over December 1955 through December 2015. Indeed, this index is a powerful predictor of future market excess returns. In-sample results show that the TECHPLS index consistently exhibits stronger predictive power than the EW index, the PC index, and 14 individual technical indicators and that its predictability is both statistically and economically significant. TECHPLS continues to perform well after I control for 14 popular macroeconomic predictor variables from Goyal and Welch (2008). In out-of-sample tests for the forecast evaluation period spanning from December 1970 to December 2015, a predictive regression forecast based on TECHPLS outperforms the prevailing average benchmark in terms of MSFE by a statistically and economically significant margin according to the CW-test statistic. The information contained in the TECHPLS-based forecast dominates the information found in forecasts based on 14 individual technical indicators. Consistently, the evidence from the Chinese equity market confirms that TECHPLS does a good job of forecasting returns based on both in-sample and out-of-sample tests, which mitigates the data-snooping concern. Furthermore, TECHPLS successfully forecasts cross-sectional stock returns, including portfolios sorted by size, BM, momentum, and industry, and generates substantial utility gains for a mean-variance investor across levels of risk aversion relative to TECHEW and TECHPC, where the gains are especially large due to better tracking of the substantial fluctuations in economic expansions. Finally, after I control for the information in popular macroeconomic predictors from the literature, TECHPLS anticipates future aggregate cash flows, suggesting that the strong ability of TECHPLS to forecast aggregate stock market returns largely stems from the cash flow channel rather than discount rate channel.