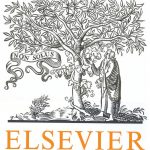
ترجمه مقاله نقش ضروری ارتباطات 6G با چشم انداز صنعت 4.0
- مبلغ: ۸۶,۰۰۰ تومان
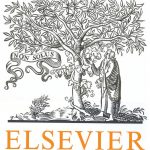
ترجمه مقاله پایداری توسعه شهری، تعدیل ساختار صنعتی و کارایی کاربری زمین
- مبلغ: ۹۱,۰۰۰ تومان
Abstract
In the era of big data, the amount of data that individuals and enterprises hold is increasing, and the efficiency and effectiveness of data analysis are increasingly demanding. Collaborative deep learning, as a machine learning framework that can share users’ data and improve learning efficiency, has drawn more and more attention and started to be applied in practical problems. In collaborative deep learning, data sharing and interaction among multi users may lead data leakage especially when data are very sensitive to the user. Therefore, how to protect the data privacy when processing collaborative deep learning becomes an important problem. In this paper, we review the current state of art researches in this field and summarize the application of privacy-preserving technologies in two phases of collaborative deep learning. Finally we discuss the future direction and trend on this problem.
V. CONCLUSION
In this paper, we first introduce the architecture of collaborative deep learning and the issue of privacy leakage. Secondly, we analyze the application of the commonly used privacy-preserving technology in the two phases of collaborative deep learning and its advantages and disadvantages. Although secure multi-party computing and homomorphic encryption can achieve a high level of privacy and accuracy, the cost is high computational and communication overhead for the users. A more practical and efficient approach is to use differential privacy, where the users insert random noise into their data before sending them to the server. However, it will reduce the accuracy of the model. Compared with traditional machine learning, many factors need to be taken into account such as the hardware performance of user equipment, transmission costs and time constraints when using privacy-preserving technology in collaborative deep learning. When organizations such as hospitals or banks that have large amounts of sensitive data act as users, homomorphic encryption technology is required to ensure the security of the model. When a large number of individuals with weak computing power act as users, differential privacy technology is required to ensure the efficiency of the model. Each privacy-preserving technology has its own characteristics, and more and more studies are currently focusing on providing a reasonable trade-off between data privacy and utility through a combination of secure multiparty computing, homomorphic encryption, and differential privacy.
With the improvement of mobile devices performance, more and more applications use collaborative deep learning to provide users with useful personalized services. Researching the privacy-preserving technology in a complex mobile environment is the next step in our work.