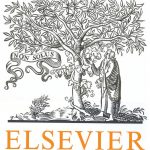
ترجمه مقاله نقش ضروری ارتباطات 6G با چشم انداز صنعت 4.0
- مبلغ: ۸۶,۰۰۰ تومان
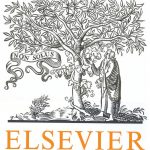
ترجمه مقاله پایداری توسعه شهری، تعدیل ساختار صنعتی و کارایی کاربری زمین
- مبلغ: ۹۱,۰۰۰ تومان
Abstract
Surface approximation with smooth functions suffers the problems of choosing the basis functions and representing non-smooth features. In this work, we introduce a sparse representation for surfaces with a set of redundant basis functions, which efficiently overcomes the overfitting artifacts. Moreover, we propose an approach of parameterization transformation, which makes the possibility to represent non-smooth features by the composition of a smooth function and a non-smooth domain optimization. We couple the sparse representation and the parameterization transformation in a global optimization to respect sharp features with smooth polynomial basis functions. Our approach is capable for approximating a wide range of surfaces with different level of sharp features. Experimental results have shown the feasibility and applicability of our proposed method in various applications.
6. Conclusion and future work
In this paper, we propose a novel surface approximation method by coupling sparse representation and parameterization optimization in a global optimization. The introduction of parameterization optimization makes it possible to represent non-smooth features by the composition of a smooth function and a nonsmooth domain optimization, and to some extent this novel idea addresses the excessive dependency on parameterization in fitting problem. Our new approach is capable for approximating a wide range of surfaces with different level of sharp features. Experimental results show its powerfulness in different applications and potential in geometric processing. Insight. The parameterization optimization aims to find a more proper domain for predefined basis functions, it is in fact learned from the input geometry. In the point view of deep learning [49], our method is a multi-layer representation with two layers. This deeper representation definitely has stronger representation ability than just one layer. So this multi-layer property illustrates well why we can obtain promising results in surface approximation. Limitation. As we introduce a more complex formulation for sparse representation, more time is needed to solve the problem as shown in Table 1. For a simple example, our algorithm has to cost 9.2 s to reach the ideal result for the upper model in Fig. 4, traditional sparse representation costs only 0.5 s. Application on higher dimension will definitely cost too much time solving it. Future work. As discussed above, we regard our work as a pioneer for representing geometry with multi layers (two in our approach), one for parameterization transformation and one for linear combination. Since we have not found a suitable formulation for the parameterization transformation (Section 3.2.1), how to set the parameterization layer is still unknown. And we believe there are still a lot of interesting and challenging problems in geometry processing that can be formulated with multi-layer representation. We would like to dig more on this novel composite idea to solve more problems.