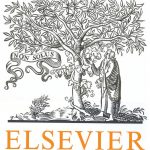
ترجمه مقاله نقش ضروری ارتباطات 6G با چشم انداز صنعت 4.0
- مبلغ: ۸۶,۰۰۰ تومان
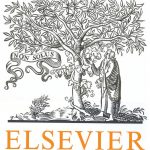
ترجمه مقاله پایداری توسعه شهری، تعدیل ساختار صنعتی و کارایی کاربری زمین
- مبلغ: ۹۱,۰۰۰ تومان
ABSTRACT
Artificial neural networks are computational approaches based on machine learning to learn and make predictions based on data, and have been applied successfully in diverse applications including structural health monitoring in civil engineering. It is difficult to optimize the weights in the neural networks that have multiple hidden layers due to the vanishing gradient issue. This paper proposes an autoencoder based framework for structural damage identification, which can support deep neural networks and be utilized to obtain optimal solutions for pattern recognition problems of highly non-linear nature, such as learning a mapping between the vibration characteristics and structural damage. Two main components are defined in the proposed framework, namely, dimensionality reduction and relationship learning. The first component is to reduce the dimensionality of the original input vector while preserving the required necessary information, and the second component is to perform the relationship learning between the features with the reduced dimensionality and the stiffness reduction parameters of the structure. Vibration characteristics, such as natural frequencies and mode shapes, are used as the input and the structural damage are considered as the output vector. A pre-training scheme is performed to train the hidden layers in the autoencoders layer by layer, and fine tuning is conducted to optimize the whole network. Numerical and experimental investigations on steel frame structures are conducted to demonstrate the accuracy and efficiency of the proposed framework, comparing with the traditional ANN methods.
Conclusion
An autoencoder based deep learning framework for structural damage identification is proposed in this paper. It can well perform the pattern recognition between the modal information, such as frequencies and mode shapes, and structural stiffness parameters. Two main components, that is, dimensionality reduction and relationship learning, are included in the proposed framework. The dimensionality reduction component utilizes an autoencoder model to compress the original input vector to obtain a robust low dimensional feature vector that preserves the necessary information through multiple hidden layers. This not only effectively removes the redundancy in the data but also keep the most useful information to serve as the input to the relationship learning component. A regression model is defined in the relationship learning component to map the compressed feature vector to the output stiffness reduction parameters. The dimensionality reduction process of the proposed framework could be applied and extended to a deeper network architecture from a complex problem. L2-weight decay is utilized to enhance the overall training process by limiting the overfitting tendency while training deep architectures. A layer-wise pretraining is performed to optimize the weights of the individual layers, and the whole network is fine-tuned using a joined optimization towards the final objective function. Numerical and experimental validations on steel frame structures are conducted and the results demonstrate the improved accuracy and efficiency of the proposed framework, comparing with the traditional ANN methods. More accurate structural damage identification results can be obtained in regards to both the locations and severities of the damages, even when both the measurement noise and uncertainty effect are considered.