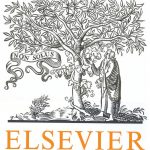
ترجمه مقاله نقش ضروری ارتباطات 6G با چشم انداز صنعت 4.0
- مبلغ: ۸۶,۰۰۰ تومان
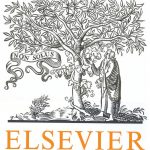
ترجمه مقاله پایداری توسعه شهری، تعدیل ساختار صنعتی و کارایی کاربری زمین
- مبلغ: ۹۱,۰۰۰ تومان
Abstract
The purpose of predictive stock price systems is to provide abnormal returns for financial market operators and serve as a basis for risk management tools. Although the Efficient Market Hypothesis (EMH) states that it is not possible to anticipate market movements consistently, the use of computationally intensive systems that employ machine learning algorithms is increasingly common in the development of stock trading mechanisms. Several studies, using daily stock prices, have presented predictive system applications trained on fixed periods without considering new model updates. In this context, this study uses a machine learning technique called Support Vector Regression (SVR) to predict stock prices for large and small capitalisations and in three different markets, employing prices with both daily and up-to-the-minute frequencies. Prediction errors are measured, and the model is compared to the random walk model proposed by the EMH. The results suggest that the SVR has predictive power, especially when using a strategy of updating the model periodically. There are also indicative results of increased predictions precision during lower volatility periods.
5. Conclusion
Developing predictive price models for the stock market is challenging, but it is an important task when building profitable financial market transaction strategies. Computationally intensive methods, using past prices, are developed to facilitate better management of market risk for investors and speculators. Of the machine learning techniques available, this study uses SVR and measures its performance on various Brazilian, American and Chinese stocks with different characteristics, for example, small cap or blue chip. The predictive variables are calculated using TA indicators on asset prices. The results show the magnitude of the mean squared errors for the three common kernels in the literature, using specific algorithm training strategies with different price frequencies of days and minutes. The results are contrasted with those of a random walk-based model.