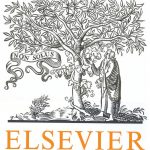
ترجمه مقاله نقش ضروری ارتباطات 6G با چشم انداز صنعت 4.0
- مبلغ: ۸۶,۰۰۰ تومان
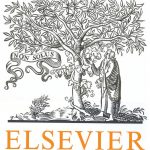
ترجمه مقاله پایداری توسعه شهری، تعدیل ساختار صنعتی و کارایی کاربری زمین
- مبلغ: ۹۱,۰۰۰ تومان
Abstract
With the rise of social web, there has also been a great concern about the quality of user-generated content on social media sites (SMSs). Deceptive comments harm users’ trust in online social media and cause financial loss to firms. Previous studies use various features and classification algorithms to detect and filter social spam on several social media platforms. However, to the best of our knowledge, previous studies have not exploited both probabilistic topic modeling and incremental learning to detect social spam on SMSs. Thus, the main contribution of this paper is design of a novel detection methodology that combines topicand user-based features to improve the effectiveness of social spam detection. The proposed methodology exploits a probabilistic generative model, namely the labeled latent Dirichlet allocation (L-LDA), for mining the latent semantics from usergenerated comments, and an incremental learning approach for tackling the changing feature space. An experiment based on a large dataset extracted from YouTube demonstrates the effectiveness of our proposed methodology, which achieves an average accuracy of 91.17 % in social spam detection. Our statistical analysis reveals that topic-based features significantly improve social spam detection, which has significant implications for business practice.
7 Conclusions
With the ubiquitous of the social web, there has been an explosive growth of usercontributed comments. Meanwhile, there has also been a growing concern about the wide spread of social spam embedded in user-contributed comments. Given the big volume of user-contributed comments on SMSs, there is a pressing need to develop novel methodologies and techniques to tackle social spam.
Previous studies use various features (e.g., user-, text, graph-, and social networkrelated attributes) and classification algorithms (e.g., Naı¨ve Bayesian and Bayesian Network) to design frameworks for detecting social spam on SMSs (e.g., Facebook, Twitter, Sina Weibo, Myspace, YouTube, and Flickr). However, to the best of our knowledge, previous studies have not exploited both probabilistic topic modeling and incremental learning for detecting social spam on SMSs. Thus, the main contributions of our research are the design and evaluation of a novel social spam methodology which is underpinned by the L-LDA model and incremental learning. More specifically, we exploit word-, topic-, and user-based features to better represent social spam and leverage incremental classifiers, such as SVM, logistic regression, perceptron, ROMMA, to enhance spam detection performance. Based on several millions of user comments posted to YouTube, our experimental results show that the proposed methodology can achieve an average accuracy of 91.17 % and an average F1-measure of 78.43 %, respectively. According to our paired t-tests, topic-based features improve the overall accuracy and precision. However, they may hurt the recall of spam detection. In contrast, user-based features enhance the recall of spam detection, but it may hurt precision.