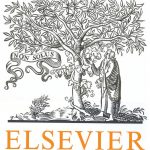
ترجمه مقاله نقش ضروری ارتباطات 6G با چشم انداز صنعت 4.0
- مبلغ: ۸۶,۰۰۰ تومان
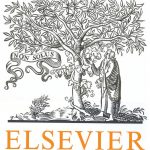
ترجمه مقاله پایداری توسعه شهری، تعدیل ساختار صنعتی و کارایی کاربری زمین
- مبلغ: ۹۱,۰۰۰ تومان
Abstract
Sparse-representation based classification (SRC) has been showing a good performance for face recognition in recent years. But SRC is not good at face recognition with low quality images (e.g., disguised, corrupted, occluded, and so on) which often appear in practical applications. To solve the problem, in this paper, we propose a novel SRC based method for face recognition with low quality images named sparse low-rank component based representation (SLCR). In SLCR, we utilize low-rank matrix recovery on the training dataset to obtain low-rank components and non-low-rank components, which are used to construct the dictionary. The new dictionary is capable of describing facial feature better, especially for low quality face samples. Furthermore, the minimum class-wise reconstruction residual is used as the recognition rule, leading to a substantial improvement on the proposed SLCR’s performance. Extensive experiments on benchmark face databases demonstrate that the proposed method is consistently superior to other sparse-representation based approaches for face recognition with low quality images.
DISCUSSION AND CONCLUSION
Our proposed SLCR consistently outperforms other sparserepresentation based methods for face recognition with low quality images. Hence, we analyze the reason why the proposed SLCR can fairly well solve the recognition problem of low quality face images. We choose an example in noise experiments (i.e., Subsection 3.3) to illustrate the procedure of the above experiments. The training samples corrupted by noise and the dictionaries reconstructed by three methods on the CMU Multi-PIE database are compared in Fig. 18. We randomly select 6 face images of the same person to reconstruct faces and extract noises. Fig. 18 (a) shows 6 face images corrupted by 20% salt-and-pepper noise, which is the dictionary of SRC exactly. Figs. 18 (b) and (c) make up the reconstructed dictionary which simply uses centroid images to capture the class specific information in SSRC. The reconstructed dictionary by the singular vectors corresponding to the largest singular value in SDR-SLR is depicted in Figs. 18(d) and (e). Similarly, Figs. 18 (f) and (g) are the reconstructed dictionary by SLCR which contains low-rank and non-low-rank component. We can see that SRC, SSRC, as well as SDR-SLR, are unable to effectively remove the noises in faces, i.e., there exists much noise in the dictionary of the three methods. On the contrary, SLCR uses low-rank matrix recovery to get low-rank component and non-low rank component which have less noise in all face images. In other words, the dictionary in SLCR not only can describe facial features well, but also can reduce the impact of noises. The better noise fitting capability of SLCR thus leads to better face recognition performance.