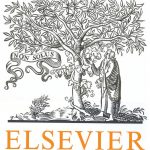
ترجمه مقاله نقش ضروری ارتباطات 6G با چشم انداز صنعت 4.0
- مبلغ: ۸۶,۰۰۰ تومان
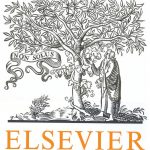
ترجمه مقاله پایداری توسعه شهری، تعدیل ساختار صنعتی و کارایی کاربری زمین
- مبلغ: ۹۱,۰۰۰ تومان
abstract
This paper proposes a big-data analytics-based approach that considers social media (Twitter) data for the identification of supply chain management issues in food industries. In particular, the proposed approach includes text analysis using a support vector machine (SVM) and hierarchical clustering with multiscale bootstrap resampling. The result of this approach included a cluster of words which could inform supply-chain (SC) decision makers about customer feedback and issues in the flow/quality of food products. A case study in the beef supply chain was analysed using the proposed approach, where three weeks of data from Twitter were used.
Conclusions
Consumers have started expressing their views on social media. Using social media data, a company may gain insight into the perception of their existing or potential consumers about their product offerings. Social media data are one of the cheapest and fastest methods to capture the viewpoint of larger audiences on a particular topic. Food is one of the most significant necessities of human life, and greatly impacts human health. In the current competitive market, consumers are searching for high-quality safe products at a minimum cost. Both positive and negative sentiments related to a particular product are crucial components for the development of a customer-centric supply chain. In this study, Twitter data were used to investigate consumer sentiments. More than one million tweets with ‘beef’ and/or ‘steak’ were collected using different keywords. Sentiment mining based on SVM and HCA with multiscale bootstrap sampling techniques was proposed for the investigation of positive and negative sentiments of the consumers, as well as for the identification of their issues/concerns regarding food products. The collected tweets were analysed to identify the main issues affecting consumer satisfaction. The root causes of these identified issues were linked to their root causes in different segments of the supply chain. As the focus of this work was to illustrate the use of the text-mining approach for social media analysis, it was therefore assumed that data from Twitter would be representative of real opinions. During the analysis of the collected tweets, it was found that the main concerns related to beef products among consumers were colour, food safety, smell, flavour, as well as the presence of foreign particles in beef products. These issues generate great disappointment among consumers. A significant number of tweets related to positive sentiments; the consumers had discovered and shared their experience about promotions, deals, and a particular combination of food and drinks with beef products. Based on these findings, a set of recommendations were prescribed for the development of a consumer-centric supply chain. However, there are certain limitations in the proposed approach. During the hierarchical clustering analysis, it was found that some of the results were not linked to the beef supply chain. These findings do not contribute towards the objective of the study, which is to develop a consumer-centric supply chain, and were therefore not described in detail. However, these results could be used for different purposes, and are a topic for future research. Moreover, other algorithms such as the latent Dirichlet algorithm may be used for the better understanding of consumer behaviours. A larger volume of tweets could be captured using Twitter Firehose instead of the streaming API, which may better represent the data. In the future, the proposed analysis could also be performed on other food supply chains, such as the lamb or pork food supply chains.