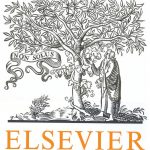
ترجمه مقاله نقش ضروری ارتباطات 6G با چشم انداز صنعت 4.0
- مبلغ: ۸۶,۰۰۰ تومان
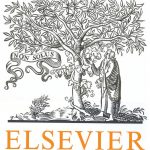
ترجمه مقاله پایداری توسعه شهری، تعدیل ساختار صنعتی و کارایی کاربری زمین
- مبلغ: ۹۱,۰۰۰ تومان
ABSTRACT
In recent years, with the raise of the neural network and deep learning, significant progress has been achieved in the field of image recognition. Convolutional Neural Network (CNN) has been widely used in multiple image recognition tasks, but the recognition accuracy still has a lot of room for improvement. In this paper, we proposed a hybrid model CNN-GRNN to improve recognition accuracy. The model uses CNN to extract multilayer image representation and it uses General Regression Neural Network (GRNN) to classify image using the extracted feature. The CNN-GRNN model replace Back propagation (BP) neural network inside CNN with GRNN to improve generalization and robustness of CNN. Furthermore, we validate our model on the Oxford-IIIT Pet Dataset database and the Keck Gesture Dataset, the experiment result indicate that our model is superior to Gray Level Co-occurrency (GLCM),HU invariant moments, CNN and CNN_SVM on small sample dataset. Our model has favorable real-time characteristic at the same time.
5. Summary
In this paper, we had introduced a hybrid model based on CNN for image classification. As CNN can extract features from images, we then use General Regression Neural Network with powerful function approximation to recognize image according to extracted representations. CNN can learn image multilayer high-dimensional feature itself which is superior to other traditional methods. And General Regression Neural Network we used can strengthen the classification and processing capacity of CNN, and it can make the model converging more quickly. Experiments show that our model is effective on image recognition. However, CNN model contains a large number of weights which leads to a large number of iterations, so it is time-consuming. Therefore, we will pay attention to optimize the training process of our model in the future.