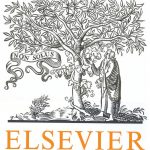
ترجمه مقاله نقش ضروری ارتباطات 6G با چشم انداز صنعت 4.0
- مبلغ: ۸۶,۰۰۰ تومان
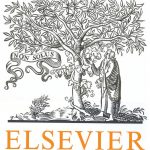
ترجمه مقاله پایداری توسعه شهری، تعدیل ساختار صنعتی و کارایی کاربری زمین
- مبلغ: ۹۱,۰۰۰ تومان
Abstract
Large volume of multi-structured and low-latency patient data are generated in healthcare services, which is challenging task to process and analyze within the Service Level Agreement (SLA). In this paper, a Parallel Semi-Naive Bayes (PSNB) based probabilistic method is used to process the healthcare big data in cloud for future health condition prediction. In order to improve the accuracy of PSNB method, a Modified Conjunctive Attribute (MCA) algorithm is proposed for reducing the dimension. Emergency condition of the patient is considered by setting a global priority among the patients and an Optimal Data Distribution (ODD) algorithm is proposed to position both batch and streaming patient data into the Spark nodes. Further, a Dynamic Job Scheduling (DJS) algorithm is designed to schedule the jobs efficiently to the most suitable nodes for processing the data taking SLA into account. Our proposed PSNB algorithm provides better accuracy of 87.8% for both batch and streaming data, which is 12.8% higher than the original NaiveBayes (NB) algorithm and can conveniently be employed in various patient monitoring applications.
Conclusion
In this work, SLA based healthcare big data analysis and computing model is proposed, where both batch and streaming patient data are analyzed in ID and ED environment. A PSNB method is proposed for healthcare Big Data analysis and a MCA algorithm is also designed for dimension reduction to improve the accuracy of PSNB algorithm. Besides, a GPS algorithm is proposed to prioritize the emergency jobs of the patients. To achieve data locality, an ODD algorithm is proposed in this paper. To improve the execution time of the prioritized jobs, a DJS algorithm is proposed that satisfies the SLA. Implementation results show that our forefront healthcare big data analytic model and proposed algorithms have outperformed in terms of accuracy and overall job completion time. The proposed model can be conveniently employed in various medical applications specifically for emergency patient data processing and analysis.