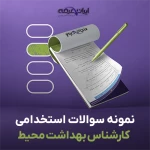
سوالات استخدامی کارشناس بهداشت محیط با جواب
- مبلغ: ۲۳۸,۰۰۰ تومان
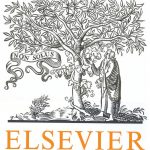
ترجمه مقاله نقش ضروری ارتباطات 6G با چشم انداز صنعت 4.0
- مبلغ: ۸۶,۰۰۰ تومان
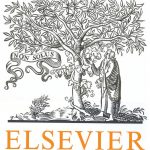
ترجمه مقاله پایداری توسعه شهری، تعدیل ساختار صنعتی و کارایی کاربری زمین
- مبلغ: ۹۱,۰۰۰ تومان
Deep Convolutional Neural Networks (DCNNs) have recently shown state of the art performance in high level vision tasks, such as image classification and object detection. This work brings together methods from DCNNs and probabilistic graphical models for addressing the task of pixel-level classification (also called ”semantic image segmentation”). We show that responses at the final layer of DCNNs are not sufficiently localized for accurate object segmentation. This is due to the very invariance properties that make DCNNs good for high level tasks. We overcome this poor localization property of deep networks by combining the responses at the final DCNN layer with a fully connected Conditional Random Field (CRF). Qualitatively, our “DeepLab” system is able to localize segment boundaries at a level of accuracy which is beyond previous methods. Quantitatively, our method sets the new state-of-art at the PASCAL VOC-2012 semantic image segmentation task, reaching 71.6% IOU accuracy in the test set. We show how these results can be obtained efficiently: Careful network re-purposing and a novel application of the ’hole’ algorithm from the wavelet community allow dense computation of neural net responses at 8 frames per second on a modern GPU.
1 INTRODUCTION
Deep Convolutional Neural Networks (DCNNs) had been the method of choice for document recognition since LeCun et al. (1998), but have only recently become the mainstream of high-level vision research. Over the past two years DCNNs have pushed the performance of computer vision systems to soaring heights on a broad array of high-level problems, including image classification (Krizhevsky et al., 2013; Sermanet et al., 2013; Simonyan & Zisserman, 2014; Szegedy et al., 2014; Papandreou et al., 2014), object detection (Girshick et al., 2014), fine-grained categorization (Zhang et al., 2014), among others. A common theme in these works is that DCNNs trained in an end-to-end manner deliver strikingly better results than systems relying on carefully engineered representations, such as SIFT or HOG features. This success can be partially attributed to the built-in invariance of DCNNs to local image transformations, which underpins their ability to learn hierarchical abstractions of data (Zeiler & Fergus, 2014). While this invariance is clearly desirable for high-level vision tasks, it can hamper low-level tasks, such as pose estimation (Chen & Yuille, 2014; Tompson et al., 2014) and semantic segmentation - where we want precise localization, rather than abstraction of spatial details.
6 DISCUSSION Our work combines ideas from deep convolutional neural networks and fully-connected conditional random fields, yielding a novel method able to produce semantically accurate predictions and detailed segmentation maps, while being computationally efficient. Our experimental results show that the proposed method significantly advances the state-of-art in the challenging PASCAL VOC 2012 semantic image segmentation task.
There are multiple aspects in our model that we intend to refine, such as fully integrating its two main components (CNN and CRF) and train the whole system in an end-to-end fashion, similar to Krahenb ¨ uhl & Koltun (2013); Chen et al. (2014); Zheng et al. (2015). We also plan to experiment ¨ with more datasets and apply our method to other sources of data such as depth maps or videos. Recently, we have pursued model training with weakly supervised annotations, in the form of bounding boxes or image-level labels (Papandreou et al., 2015).
At a higher level, our work lies in the intersection of convolutional neural networks and probabilistic graphical models. We plan to further investigate the interplay of these two powerful classes of methods and explore their synergistic potential for solving challenging computer vision tasks.