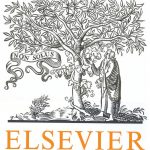
ترجمه مقاله نقش ضروری ارتباطات 6G با چشم انداز صنعت 4.0
- مبلغ: ۸۶,۰۰۰ تومان
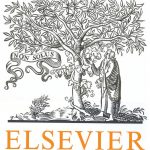
ترجمه مقاله پایداری توسعه شهری، تعدیل ساختار صنعتی و کارایی کاربری زمین
- مبلغ: ۹۱,۰۰۰ تومان
abstract
A novel transfer support vector machine called TSVM-GP with group probabilities is proposed for the scenarios where plenty of labeled data in the source domain and the group probabilities of unlabeled data in the target domain are available. TSVM-GP integrates a transfer term and group probabilities into the support vector machine (SVM) to improve the classification accuracy. In order to reduce the high computational complexity of TSVM-GP, the scalable version of TSVM-GP called scalable transfer support vector machine with group probabilities (STSVM-GP) is further developed by selecting the representative set of the training samples as the training data in the source domain. Experimental results on synthetic datasets as well as several real-world datasets show the effectiveness of the proposed classifiers, and especially STSVM-GP is very feasible for large scale transfer datasets.
6. Conclusions
Designing the classifiers from datasets with group probabilities is an important learning task for practical applications, as well as for scalable datasets. In view of this, we propose a transfer support vector machine with group probabilities (TSVM-GP) by incorporating additional group probabilities into the transfer learning framework. Furthermore, in order to make TSVM-GP scalable to large scale transfer datasets, a scalable transfer support vector machine with group probabilities (STSVM-GP) is proposed by using the representative set of the source domain as the new training set and then utilize them for transfer learning with group probability. The effectiveness of the classifiers is demonstrated using several datasets from the real-world classification datasets as well as using the synthetic datasets. Although the proposed TSVM-GP and STSVM-GP have shown promising performance, there are still many aspects that deserve further investigation. For example, how to further reduce the computation in proposed classifiers by using more efficient QP solver is a research topic worth to be studied. Furthermore, how to develop a robust classifier for noisy data with group probabilities is also worth to be studied.