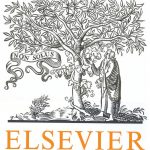
ترجمه مقاله نقش ضروری ارتباطات 6G با چشم انداز صنعت 4.0
- مبلغ: ۸۶,۰۰۰ تومان
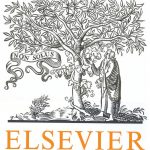
ترجمه مقاله پایداری توسعه شهری، تعدیل ساختار صنعتی و کارایی کاربری زمین
- مبلغ: ۹۱,۰۰۰ تومان
Abstract
In the construction of an artificial neural network (ANN) a proper data splitting of the available samples plays a mayor role in the training process. This selection of subsets for training, testing and validation affects the generalization ability of the neural network. Also the number of samples has an impact in the time required for the design of the ANN and the training. This paper introduces an efficient and simple method for reducing the set of samples used for training a neural network. The method reduces the required time to calculate the network coefficients, while keeping the diversity and avoiding overtraining the ANN due the presence of similar samples. The proposed method is based on the calculation of the angle between two vectors, each one representing one input of the neural network. When the angle formed among samples is smaller than a defined threshold only one input is accepted for the training. The accepted inputs are scattered throughout the sample space. Tidal records are used to demonstrate the proposed method. The results of a cross-validation show that with few inputs the quality of the outputs is not accurate and depends on the selection of the first sample, but as the number of inputs increases the accuracy is improved and differences among the scenarios with a different starting sample have and important reduction. A comparison with the K-means clustering algorithm shows that for this application the proposed method with a smaller number of samples are producing a more accurate network.
5. Conclusions
The angle between vectors is an easy method to select samples that show spreading in the space of the inputs. The proposed method enables to reduce the redundancy of information, avoiding the use of two or more samples that are similar to each other. As the selected samples are in general those that differ more from others, the resulting training dataset contains a higher diversity and is able to produce an ANN with a similar performance than networks trained with more inputs. The reduced number of samples has a clear advantage to speed-up the training process. This is relevant in the design of the architecture of a new ANN where the number of input nodes and hidden layers are still to be determined and several scenarios need to be tested to find an optimal configuration. After the architecture is defined, in some cases it is possible to increase the number of samples for the final model if still smaller errors can be obtained in a reasonable time. The proposed method is more efficient capturing extreme values than the K-means. When comparing with the clustering algorithm K-means, the proposed method is clearly providing not only an smaller dataset (with its great benefit in reducing computational time) but also an smaller RMSE.