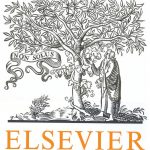
ترجمه مقاله نقش ضروری ارتباطات 6G با چشم انداز صنعت 4.0
- مبلغ: ۸۶,۰۰۰ تومان
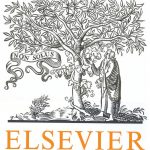
ترجمه مقاله پایداری توسعه شهری، تعدیل ساختار صنعتی و کارایی کاربری زمین
- مبلغ: ۹۱,۰۰۰ تومان
abstract
In big data fields, with increasing computing capability, artificial neural networks have shown great strength in solving data classification and regression problems. The traditional training of neural networks depends generally on the error back propagation method to iteratively tune all the parameters. When the number of hidden layers increases, this kind of training has many problems such as slow convergence, time consuming, and local minima. To avoid these problems, neural networks with random weights (NNRW) are proposed in which the weights between the hidden layer and input layer are randomly selected and the weights between the output layer and hidden layer are obtained analytically. Researchers have shown that NNRW has much lower training complexity in comparison with the traditional training of feed-forward neural networks. This paper objectively reviews the advantages and disadvantages of NNRW model, tries to reveal the essence of NNRW, gives our comments and remarks on NNRW, and provides some useful guidelines for users to choose a mechanism to train a feed-forward neural network.
4. Concluding remarks
In this paper, we present a thorough survey on the evolvement of feed-forward neural networks with random weights (NNRW), especially its applications in deep learning. In NNRW, due to the weights and the threshold of hidden layer are randomly selected and the weights of output layer are obtained analytically, NNRW can achieve much faster learning speed than BP-based methods. As described above, NNRW have been widely applied to many applications. Traditional deep learning has produced lots of breakthrough results in recent years.
However, it suffers from several notorious problems, such as numerous parameters that need to be tuned, high requirements for computing resources, low convergence rate, and high computational complexity, etc. This paper has shown that the combination of traditional deep learning and NNRW can greatly improve the computing efficiency of deep learning. However, there are still several open problems need to be addressed, such as how to determine the randomization range and the type of distribution of the hidden weights? It is well known that, the randomization range and the type of distribution of the hidden weights have significant impact on the performance of NNRW.However, there is no clear criterion to guide the selection of the hidden weights. In most cases, the authors directly set the randomization range to an empirical range (i.e., [−1, 1]). But this range can not guarantee the optimal performance of NNRW [15]. In addition, NNRW have shown good generalization performance on the problems with higher noise, how to prove it in theory and estimate the oscillation bound of the generalization performance are not clear.