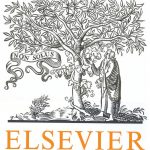
ترجمه مقاله نقش ضروری ارتباطات 6G با چشم انداز صنعت 4.0
- مبلغ: ۸۶,۰۰۰ تومان
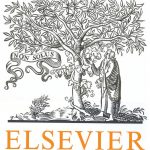
ترجمه مقاله پایداری توسعه شهری، تعدیل ساختار صنعتی و کارایی کاربری زمین
- مبلغ: ۹۱,۰۰۰ تومان
Abstract
In the last few years, deep learning has led to very good performance on a variety of problems, such as visual recognition, speech recognition and natural language processing. Among different types of deep neural networks, convolutional neural networks have been most extensively studied. Leveraging on the rapid growth in the amount of the annotated data and the great improvements in the strengths of graphics processor units, the research on convolutional neural networks has been emerged swiftly and achieved stateof-the-art results on various tasks. In this paper, we provide a broad survey of the recent advances in convolutional neural networks. We detailize the improvements of CNN on different aspects, including layer design, activation function, loss function, regularization, optimization and fast computation. Besides, we also introduce various applications of convolutional neural networks in computer vision, speech and natural language processing.
6. Conclusions and Outlook
Deep CNNs have made breakthroughs in processing image, video, speech and text. In this paper, we have given an extensive survey on recent advances of CNNs. We have discussed the improvements of CNN on different aspects, namely, layer design, activation function, loss function, regularization, optimization and fast computation. Beyond surveying the advances of each aspect of CNN, we have also introduced the application of CNN on many tasks, including image classification, object detection, object tracking, pose estimation, text detection, visual saliency detection, action recognition, scene labeling, speech and natural language processing.
Although CNNs have achieved great success in experimental evaluations, there are still lots of issues that deserve further investigation. Firstly, since the recent CNNs are becoming deeper and deeper, they require large-scale dataset and massive computing power for training. Manually collecting labeled dataset requires huge amounts of human efforts. Thus, it is desired to explore unsupervised learning of CNNs. Meanwhile, to speed up training procedure, although there are already some asynchronous SGD algorithms which have shown promising result by using CPU and GPU clusters, it is still worth to develop effective and scalable parallel training algorithms. At testing time, these deep models are highly memory demanding and timeconsuming, which makes them not suitable to be deployed on mobile platforms that have limited resources. It is important to investigate how to reduce the complexity and obtain fast-to-execute models without loss of accuracy.
Furthermore, one major barrier for applying CNN on a new task is that it requires considerable skill and experience to select suitable hyperparameters such as the learning rate, kernel sizes of convolutional filters, the number of layers etc. These hyper-parameters have internal dependencies which make them particularly expensive for tuning. Recent works have shown that there exists a big room to improve current optimization techniques for learning deep CNN architectures [12, 42, 316].
Finally, the solid theory of CNNs is still lacking. Current CNN model works very well for various applications. However, we do not even know why and how it works essentially. It is desirable to make more efforts on investigating the fundamental principles of CNNs. Meanwhile, it is also worth exploring how to leverage natural visual perception mechanism to further improve the design of CNN. We hope that this paper not only provides a better understanding of CNNs but also facilitates future research activities and application developments in the field of CNNs.