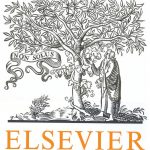
ترجمه مقاله نقش ضروری ارتباطات 6G با چشم انداز صنعت 4.0
- مبلغ: ۸۶,۰۰۰ تومان
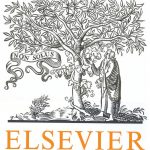
ترجمه مقاله پایداری توسعه شهری، تعدیل ساختار صنعتی و کارایی کاربری زمین
- مبلغ: ۹۱,۰۰۰ تومان
Abstract
Reliable data delivery in the Internet of Things (IoT) is very important in order to provide IoT-based services with the required quality. However, IoT data delivery may not be successful for different reasons, such as connection errors, external attacks, or sensing errors. This results in data incompleteness, which decreases the performance of IoT applications. In particular, the recovery of missing data among the massive sensed data of the IoT is so important that it should be solved. In this paper, we propose a probabilistic method to recover missing (incomplete) data from IoT sensors by utilizing data from related sensors. The main idea of the proposed method is to perform probabilistic matrix factorization (PMF) within the preliminary assigned group of sensors. Unlike previous PMF approaches, the proposed model measures the similarity in data among neighboring sensors and splits them into different clusters with a K-means algorithm. Simulation results show that the proposed PMF model with clustering outperforms support vector machine (SVM) and deep neural network (DNN) algorithms in terms of accuracy and root mean square error. By using normalized datasets, PMF shows faster execution time than SVM, and almost the same execution time as the DNN method. This proposed incomplete data–recovery approach is a promising alternative to traditional DNN and SVM methods for IoT telemetry applications.
CONCLUSION
One of the problems in realizing the IoT is missing sensor data values that are crucial for decision-making processes in different applications. The missing sensor values are necessary for applications that require them as data input. By clustering related sensors and using probabilistic matrix factorization, this paper has shown how to recover massive amounts of missing sensor data values. Our results show that by minimizing RMSE using the PMF method inside a cluster of sensor nodes, missing sensor values can be recovered more efficiently than with other methods, such as SVM and DNN. The SVM and DNN methods have less accuracy and higher RMSE values, compared to the PMF method, due to loss of precision for continuous datasets. The PMF model applied inside closely related sensors shows better accuracy and lower RMSE results than other methods. As the cluster size gets smaller (for better correlation of measurements among sensors), accuracy also becomes better compared to larger clusters. The reason is that a larger cluster size contains more sensors, and the correlation between measurements is not good among all sensors.