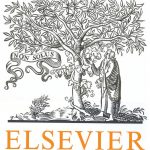
ترجمه مقاله نقش ضروری ارتباطات 6G با چشم انداز صنعت 4.0
- مبلغ: ۸۶,۰۰۰ تومان
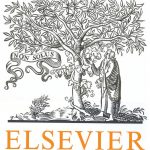
ترجمه مقاله پایداری توسعه شهری، تعدیل ساختار صنعتی و کارایی کاربری زمین
- مبلغ: ۹۱,۰۰۰ تومان
abstract
With the fast development of cloud computing, more and more data storage and computation are moved from the local to the cloud, especially the applications of machine learning and data analytics. However, the cloud servers are run by a third party and cannot be fully trusted by users. As a result, how to perform privacy-preserving machine learning over cloud data from different data providers becomes a challenge. Therefore, in this paper, we propose a novel scheme that protects the data sets of different providers and the data sets of cloud. To protect the privacy requirement of different providers, we use public-key encryption with a double decryption algorithm (DD-PKE) to encrypt their data sets with different public keys. To protect the privacy of data sets on the cloud, we use ϵ-differential privacy. Furthermore, the noises for the ϵ-differential privacy are added by the cloud server, instead of data providers, for different data analytics. Our scheme is proven to be secure in the security model. The experiments also demonstrate the efficiency of our protocol with different classical machine learning algorithms.
Conclusion and future work
In this paper, we proposed PMLM, a scheme for privacypreserving machine learning under multiple keys, which allows multiple data providers to outsource encrypted data sets to a cloud server for data storing and processing. In our work, the cloud server can add different statistical noises to the outsourced data sets according to the different queries of the data analyst, which is different from existing works (i.e., data providers add statistical noise by themselves). Our work is mainly based on DDPKE cryptosystem Π1 and ϵ-DP, which can be proven to achieve the goal of outsourced computation on multi-party’s data sets without privacy leakage in the random oracle model. Many important works have shown that differential privacy is an effective and useful tool for data privacy calculations. As a further research work, we hope that our PMLM scheme will be useful in both the application domain and theory domain of privacypreserving machine learning.