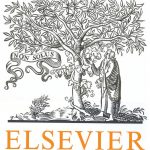
ترجمه مقاله نقش ضروری ارتباطات 6G با چشم انداز صنعت 4.0
- مبلغ: ۸۶,۰۰۰ تومان
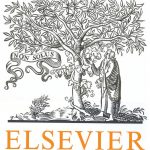
ترجمه مقاله پایداری توسعه شهری، تعدیل ساختار صنعتی و کارایی کاربری زمین
- مبلغ: ۹۱,۰۰۰ تومان
abstract
In this paper, we compute flash estimates of Finnish monthly economic activity using firmlevel data. We use a two-step procedure where the common factors extracted from the firm-level data are subsequently used as predictors in nowcasting regressions. The results show that large firm-level datasets are useful for predicting aggregate economic activity in a timely fashion. The proposed factor-based nowcasting model leads to a superior outof-sample nowcasting performance relative to the benchmark autoregressive model, even for early nowcasts. Moreover, we find that the quarterly GDP flash estimates that we construct provide a useful real-time alternative to the current official estimates, without any substantial loss of nowcasting accuracy. © 2015 Published by Elsevier B.V. on behalf of International Institute of Forecasters.
5. Conclusions
In this study, we have used a large dataset of Finnish firm-level turnovers to compute factors which are in turn included in a predictive regression for nowcasting monthly economic activity. We compute the factors using two methods. In the first method, we simply eliminate the firms that present jagged edges or missing values, thus ensuring that the turnover dataset is balanced, and use a simple principal component estimator to extract the factors. We call this routine a balanced method. In our other method, we perform missing value imputation using the factor model and the regularized EM algorithm proposed by Josse and Husson (2012a). This method allows us to use all of the firms in the dataset, but is also computationally more intensive than the balanced method.