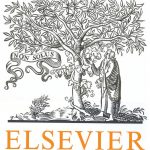
ترجمه مقاله نقش ضروری ارتباطات 6G با چشم انداز صنعت 4.0
- مبلغ: ۸۶,۰۰۰ تومان
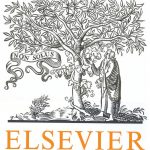
ترجمه مقاله پایداری توسعه شهری، تعدیل ساختار صنعتی و کارایی کاربری زمین
- مبلغ: ۹۱,۰۰۰ تومان
Abstract
During the last years, there is increasing interest in analyzing social networks and modeling their dynamics at different scales. This work focuses on predicting the future form of communities, which represent the mesoscale structure of networks, while the communities arise as a result of user interaction. We employ several structural and temporal features to represent communities, along with their past form, that are used to formulate a supervised learning task to predict whether a community will continue as currently is, shrink, grow or completely disappear. To test our methodology, we created a reallife social network dataset consisting of an excerpt of posts from the Mathematics Stack Exchange Q&A site. In the experiments, special care is taken in handling the class imbalance in the dataset and in investigating how the past evolutions of a community affect predictions.
V. CONCLUSIONS
This work aimed at predicting the evolution of communities that are formed in social networks as a result of user interaction, using a mixture of structural and temporal features. Four types of evolution that commonly arise in social networks were examined, namely the continuation, growth, shrinkage and dissolution of communities. We presented a framework that incorporates all necessary steps for building a predictive model to infer community evolution. These steps are: segmentation into timeframes, detection and tracking of communities, calculation of communities’ features and classifier training. We performed experiments using real-life social network data acquired from the Mathematics Stack Exchange Q&A site. Experiments demonstrated that prediction accuracy improves when temporal features are used on top of the structural ones. Also, the extent of past evolutions of a community considered (i.e., the number of ancestors) affects predictions and using four ancestors gave the best results in our dataset. It seems that the past of a community encapsulates information about its future evolution and can help in improving predictions, if we do not go too far back in time.
Future work will focus on the prediction of other types of community evolution, such as merges and splits where there is no one-to-one correspondence between communities as they evolve. The incorporation of other types of features in order to improve predictions, such as features derived from the text posted by social network users (e.g., topics of discussion and sentiment) and features related to the context of a particular social network (e.g., reputation in the Mathematics Stack Exchange site and hashtags in Twitter), could be also examined. In addition, using other classifiers, apart from SVMs, for predictions and performing tests with more datasets, as well as comparing our approach to existing ones from the literature, such as [14], is in our plans. Moreover, finding the optimal timeframes for splitting the data stream of a social network poses an interesting problem itself. Such optimal timeframes would contribute in a more accurate detection of communities and subsequently their tracking and prediction.