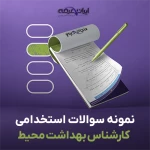
سوالات استخدامی کارشناس بهداشت محیط با جواب
- مبلغ: ۲۳۸,۰۰۰ تومان
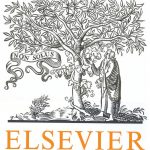
ترجمه مقاله نقش ضروری ارتباطات 6G با چشم انداز صنعت 4.0
- مبلغ: ۸۶,۰۰۰ تومان
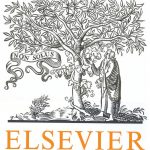
ترجمه مقاله پایداری توسعه شهری، تعدیل ساختار صنعتی و کارایی کاربری زمین
- مبلغ: ۹۱,۰۰۰ تومان
Real-life problems usually include conflicting objectives. Solving multi-objective problems (i.e., obtaining the complete efficient set and the corresponding Pareto-front) via exact methods is in many cases nearly intractable. In order to cope with those problems, several (meta) heuristic procedures have been developed during the last decade whose aim is to obtain a good discrete approximation of the Pareto-front. In this vein, a new multi-objective evolutionary algorithm, called FEMOEA, which can be applied to many nonlinear multi-objective optimization problems, has recently been proposed. Through a comparison with an exact interval branch-and-bound algorithm, it has been shown that FEMOEA provides very good approximations of the Pareto-front. Furthermore, it has been compared to the reference algorithms NSGA-II, SPEA2 and MOEA/D. Comprehensive computational studies have shown that, among the studied algorithms, FEMOEA was the one providing, on average, the best results for all the quality indicators analyzed. However, when the set approximating the Pareto-front must have many points (because a high precision is required), the computational time needed by FEMOEA may not be negligible at all. Furthermore, the memory requirements needed by the algorithm when solving those instances may be so high that the available memory may not be enough. In those cases, parallelizing the algorithm and running it in a parallel architecture may be the best way forward. In this work, a parallelization of FEMOEA, called FEMOEA-Paral, is presented. To show its applicability, a bi-objective competitive facility location and design problem is solved. The results show that FEMOEA-Paral is able to maintain the effectiveness of the sequential version and this by reducing the computational costs. Furthermore, the parallel version shows good scalability. The efficiency results have been analyzed by means of a profiling and tracing toolkit for performance analysis.
1. Introduction
Multi-objective optimization problems are ubiquitous. Many real-life problems require taking several conflicting points of view into account [2,3,11,12,20,29]. Thus, general multi-objective optimization algorithms able to cope with those hardto-solve optimization problems are required.
5. Conclusions
To deal with hard-to-solve multi-objective optimization problems, as most competitive location problems are, a parallel version of FEMOEA, called FEMOEA-Paral, has been developed and analyzed. A comprehensive computational study has shown that FEMOEA-Paral maintains the effectiveness of the sequential version, i.e. both versions approximate the Pareto-front with 100% success in all the instances, their hypervolume values are always included in the interval provided by iB&B and they both obtain similar hypervolume values for any particular instance. The maintenance of the effectiveness values is made possible thanks to the implemented selection procedure, which allows us to concurrently choose the most preferable solutions. The efficiency of the parallel version has also been tested. The distribution of the computational load carried out by FEMEOA-Paral allows us to highly accelerate the sequential computational times, in such a way that FEMOEA-Paral has been able to obtain super efficiency values. Additionally, the scalability of the parallel version has also been shown by solving instances with a larger computational burden.
Efficiency results have been analyzed by using TAU. It can be concluded that the parallelization overhead increases with the number of processors. Furthermore, it was found that whereas the distribution of the population among the processors saves CPU time as compared to the sequential version (the number of cache misses is smaller), the opposite holds with the selection procedure, which acts as a bottleneck in the algorithm. New selection procedures, able to maintain the effectiveness, but without causing load imbalance, should be researched. Additionally, methods to reduce the cache misses for the sequential as well as for the parallel version should be studied. An analytical study should also be carried out to try to characterize the improvements that can be achieved with the parallelization
1. مقدمه
مسائل بهینه سازی چندهدفه, همه جا وجود دارند. بسیاری از مسائل زندگی واقعی به اتخاذ چندین دیدگاه متناقض نیاز دارند [2,3,11,12,20,29]. بنابراین, الگوریتم های بهینه سازی چندهدفه کلی قادر به حل مسائل بهینه سازی دارای مشکل در حل کردن هستند.
5. نتایج
برای حل مسائل بهینه سازی دارای مشکل حل کردن و چندهدفه, همانند بسیاری از مسائل موقعیت یابی رقابتی, یک نسخه موازی از FEMOEA به نام FEMOEA-Paral توسعه و تحلیل شده است. یک مطالعه محاسباتی جامع نشان داده است که FEMOEA-Paral, اثربخشی نسخه ترتیبی را حفظ می کند, یعنی هر دو نسخه, پارتو-جلو را با 100% موفقیت در تمام نمونه ها تخمین می زنند, مقادیر هایپروولوم آنها همیشه در بازه فراهم شده توسط iB&B گنجانده می شوند و آنها هر دو, مقادیر هایپروولوم مشابه را برای هر نمونه خاص به دست می آورند. نگهداری مقادیر اثربخشی به لطف رویه انتخاب پیاده سازی شده ممکن می شود که به طور همزمان انتخاب ارجح ترین راه حل ها را ممکن می سازد. بازده نسخه موازی نیز تست شده است. توزیع بار محاسباتی انجام شده توسط FEMEOA-Paral تسریع زیاد زمان های محاسباتی ترتیبی را برای ما میسر می سازد, به گونه ای که FEMOEA-Paral قادر به کسب مقادیر بازده بسیار بالاست. علاوه بر این, مقیاس پذیری نسخه موازی نیز توسط حل نمونه ها با بار محاسباتی بیشتر نشان داده شده است.
نتایج بهره وری با استفاده از TAU تحلیل شده است. می توان نتیجه گرفت که سربار موازی سازی با تعداد پردازنده افزایش می یابد. علاوه بر این، مشخص شد که در حالی که توزیع جمعیت در میان پردازنده ها موجب صرفه جویی در زمان CPU نسبت به نسخه های ترتیبی می شود (تعداد خطاهای کش کوچکتر است)، مخالف آن با رویه انتخاب برقرار است، که به عنوان یک تنگنا در الگوریتم عمل می کند . روش های انتخاب جدید، قادر به حفظ اثربخشی هستند، اما بدون ایجاد عدم تعادل بار، باید مورد بررسی قرار گیرند. علاوه بر این، روش ها برای کاهش خطاهای کش برای نسخه ترتیبی و همچنین برای نسخه موازی باید مطالعه شوند.. یک مطالعه تحلیلی نیز باید برای توصیف بهبودی که می توان با موازی سازی کسب نمود انجام شود.