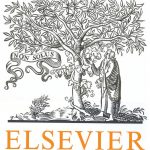
ترجمه مقاله نقش ضروری ارتباطات 6G با چشم انداز صنعت 4.0
- مبلغ: ۸۶,۰۰۰ تومان
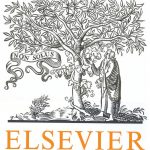
ترجمه مقاله پایداری توسعه شهری، تعدیل ساختار صنعتی و کارایی کاربری زمین
- مبلغ: ۹۱,۰۰۰ تومان
Abstract
A futures trading evaluation system is used to help investors analyze their trading history and find out the root cause of profit and loss, so that investors can learn from their past and make better decisions in the future. To analyze trading history of investors, the system processes a large volume of transaction data to calculate key performance indicators (KPI) as well as time series behavior patterns, and concludes some recommendations with the help of an expert knowledge base. This work is based on our early work of parallel techniques for large data analysis for futures trading evaluation service. In our early work, we have used the query rewriting technique to avoid joining between fact table and dimension table for OLAP aggregation queries, and used a data driven shared scanning of data method to compute KPIs for one customer. However, the query rewriting technique cannot eliminate joining for queries which aggregate on an intermediate level of the hierarchy of a dimensional table, so we propose a segmented bit encoding of dimensional table method which can eliminate the joining operation when the query aggregates on any level of the hierarchy of any dimensional table. Furthermore, our previous method perform badly when concurrency is high, so we propose an inter customer data scan sharing scheme to improve system performance in highly concurrent situations. We present our new experimental results.
5. Related works and discussion
Segmented bit encoding of dimensional information has borrowed ideas from universal relation [3]. However, our scheme doesn’t put all dimension information but hierarchy information into the fact table, thus it is more space-saving compared with universal relation. Then the hierarchical information is used by most aggregation queries in our applications. IBM has proposed BLINK [4] prototype to pre join dimension tables and the fact table to form a single wide table, which results in much simpler query processing. Table scanning is parallelized and constant query response time is achieved. De-normalization of data leads to data redundancy. Our scheme does not incur as much data redundancy as BLINK. In the domain of scientific research, simulation, internet, e-commerce, as well as the financial data analysis areas discussed in the paper, it is witnessed that the data volume is growing rapidly [5]. Traditional data warehouse technology could not deal with the rapid exploding data effectively. Google has brought forward the MapReduce technology, which is a parallel computing software framework [6] to deal with very large data sets. In Google, more than 20 PB of data is processed every day using MapReduce. MapReduce has demonstrated its power in the area of big data processing [7].