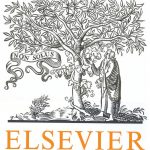
ترجمه مقاله نقش ضروری ارتباطات 6G با چشم انداز صنعت 4.0
- مبلغ: ۸۶,۰۰۰ تومان
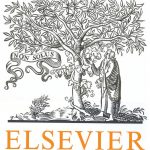
ترجمه مقاله پایداری توسعه شهری، تعدیل ساختار صنعتی و کارایی کاربری زمین
- مبلغ: ۹۱,۰۰۰ تومان
Abstract:
With the increasing number of users on Social Networking Service (SNS), the Internet of knowledge shared on it is also increasing. Given such enhancement of Internet of knowledge on SNS, the probability of spreading spammers on it is also increasing day by day. Several traditional machine-learning methods, such as support vector machines and naïve Bayes, have been proposed to detect spammers on SNS. Note, however, that these methods are not efficient due to some issues, such as lower generalization performance and higher training time. An Extreme Learning Machine (ELM) is an efficient classification method that can provide good generalization performance at higher training speed. Nonetheless, it suffers from overfitting and ill-posed problem that can degrade its generalization performance. In this paper, we propose a Bagging ELM-based spammer detection framework that identifies spammers in SNSs with the help of multiple ELMs that we combined using the bagging method. We constructed a labeled dataset of the two most prominent SNSs -- Twitter and Facebook -- to evaluate the performance of our framework. The evaluation results show that our framework obtained higher generalization performance rate of 99.01% for the Twitter dataset and 99.02 % for the Facebook datasets, while required a lower training time of 1.17s and 1.10s, respectively.
5. Conclusion
In this paper, we proposed a Bagging ELM-based spammer detection framework for SNSs. Our proposed framework has three major contributions in this area. First, it identifies account- and object-specific features to facilitate spammer detection in SNSs. Second, it constructs a novel dataset of the two most popular SNSs, i.e., Twitter and Facebook. Finally, it introduces a Bagging ELM classifier and applies this classifier to the dataset that we constructed from Twitter and Facebook. Our experiments and comparison with other traditional classifiers show that our framework is able to achieve much better generalization performance than other existing frameworks. Our framework achieved average accuracy rate of 99.01 % for the Twitter dataset and 99.02 % for the Facebook dataset while requiring shorter training time of 1.17s and 1.10s, respectively. Note, however, that the performance result of the framework relies on the labeled dataset, which typically needs considerable labor cost and time. Furthermore, manually labeling the process to obtain the labeled dataset suffers from inaccurate result due to individual bias. To address the issue of labeled dataset, our framework can be enhanced by using semi-supervised learning with ELM. The semi-supervised ELM can support the use of easily acquired unlabeled dataset and provide good generalization performance at higher speed.