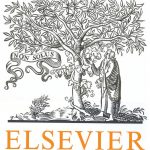
ترجمه مقاله نقش ضروری ارتباطات 6G با چشم انداز صنعت 4.0
- مبلغ: ۸۶,۰۰۰ تومان
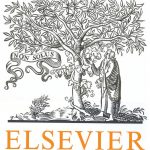
ترجمه مقاله پایداری توسعه شهری، تعدیل ساختار صنعتی و کارایی کاربری زمین
- مبلغ: ۹۱,۰۰۰ تومان
Abstract
Brain is the strongest part of the human body which consists of the number of neurons. Electrical activity of the brain can be measured using many techniques in which EEG is widely used. Any change in electrical signal will define a particular abnormality in human. This paper, suggest a algorithm for the EEG signal analysis for the detection of seizure using wavelet transform and statistical parameters. Data set consists of two sampling rates, one with 128Hz and another with sampling rate of 1024Hz. Feature extraction was done using discrete wavelet transform. Once a feature extraction is done the data will be given to a neural network for the classification. A multilayered neural network was used classify seizure and normal person. The proposed algorithm is tested on 23 data sets. Classification accuracy of 100% has been achieved for the sampling rate of 1024 and 85% for the data with sampling rate of 128. Total system accuracy achieved is 92.5%.
VI. CONCLUSION
In this paper, two database have been used, one with 128Hz and another is with 1024Hz. Feature extraction was done using DWT method and statistical parameter were calculated on wavelet coefficients. For classification purposes, three different sets have been considered. Considering all the bands at once, considering only three bands at a time and finally considering the individual band. From the Table I, II conclude that for the sampling rate of 1024 Hz results are more accurate. This paper also concludes that the accuracy is less when sampling rate is less. But when sampling rate is increased decomposition level increases which in turn increases the processing time.