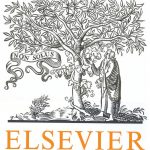
ترجمه مقاله نقش ضروری ارتباطات 6G با چشم انداز صنعت 4.0
- مبلغ: ۸۶,۰۰۰ تومان
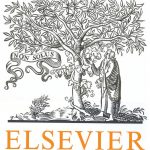
ترجمه مقاله پایداری توسعه شهری، تعدیل ساختار صنعتی و کارایی کاربری زمین
- مبلغ: ۹۱,۰۰۰ تومان
abstract
We examine the situation in which hourly data are available for designing advertisingresponse models, whereas managerial decision-making can concern hourly, daily or weekly intervals. A key notion is that models for higher frequency data require the intra-seasonal heterogeneity to be addressed, while models for lower frequency data are much simpler. We use three large, actual real-life datasets to analyze the relevance of these additional efforts for managerial interpretation and for the out-of-sample forecast accuracy at various frequencies. © 2016 International Institute of Forecasters. Published by Elsevier B.V. All rights reserved.
8. Conclusion and discussion
This paper presents detailed estimation and forecasting results for three large datasets that contain high frequency information on advertising and responses. We cannot claim to have discovered a generalizing principle for these three cases, but we do find that properly-specified models for high frequency data can achieve appropriate forecast accuracies, and compare well with models for temporally aggregated data. Therefore, the main argument in this paper is that models for higher frequency data probably do not have constant parameters, and that a forecaster should take care of that. This intra-seasonal heterogeneity can be captured in a two-level regression model (LMM). In our case, a model for hourly data requires the variation across those hours to be modeled, and we advocate using the LMM for this purpose. Aggregating the hourly data to weekly data reduces this variation. Using three real-life cases, we have shown that, when done properly, models for higher frequency data are more useful than forecasts from models for aggregated data in terms of managerial use, and equally useful in terms of forecasting such aggregated data.