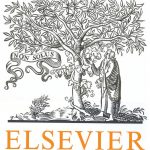
ترجمه مقاله نقش ضروری ارتباطات 6G با چشم انداز صنعت 4.0
- مبلغ: ۸۶,۰۰۰ تومان
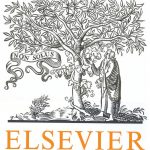
ترجمه مقاله پایداری توسعه شهری، تعدیل ساختار صنعتی و کارایی کاربری زمین
- مبلغ: ۹۱,۰۰۰ تومان
Abstract
In order to achieve higher fidelity (i.e., higher accuracy) in the model predictions, the solution models employed in geological and/or geotechnical engineering are becoming complex and sophisticated. However, simple and robust models are preferred by engineers in practice. Thus, a dilemma exists between the choice of a complex model for fidelity and that of a simple model for high robustness (i.e., lower variation in the discrepancy between model prediction and observation). This issue becomes more profound when model parameters exhibit uncertainty, which is quite common in geological and geotechnical problems. In this paper, we examine the issue of model selection in the face of uncertainty with three problems: the selection of the order of polynomial fit (i.e., lower order vs. higher order) in the development of data-driven empirical model, the selection of the level of sophistication (i.e., random variable vs. random field) in the probabilistic characterization of the detrended soil property, and the selection of the level of complexity (i.e., simple vs. complex) of the soil constitutive model in numerical modelling. The results illustrate that although the complex and sophisticated models could yield predictions that are more accurate, the simple models might yield predictions that are more robust. This paper provides an insight regarding the question, “Does a complex model always outperform a simple model?”
5. Concluding Remarks
This paper presented a preliminary study on the selection of solution models in the face of uncertainty (in the model parameters) by means of three representative geotechnical problems. The first problem considers the selection of the order of the polynomial fit in the development of data-driven empirical models, the second problem considers the selection between the random variable and the random field in the probabilistic characterization of a soil property at a site, and the third problem considers the selection of soil constitutive models in the numerical modelling of a braced excavation. The following conclusions are drawn based upon the results presented: (1) Due to the limited availability of site-specific data, and the existence of test error and inherent variability in natural deposits, the model parameters of the geotechnical model could not be characterized with certainty, which tends to result in a significant uncertainty in the predicted system performance. Because of the uncertainty in the model parameters, a complex model may be more accurate but less robust. Further, the uncertainty in the model parameters of the complex model could be more difficult to be characterized, as a larger number of model parameters are involved. Thus, a complex model does not necessarily indicate a better model; instead, a simple model might outperform a complex model in the face of uncertainty in the model parameters. (2) In the development of data-driven empirical models in geological and/or geotechnical engineering, the fidelity could be guaranteed by adopting a higher-order polynomial fit. Even so, discrepancies between model predictions and field observations always exist; and as such, model parameters should be characterized as uncertain variables and the intended system performance should be evaluated in a probabilistic manner. The robustness of a model, which can be indicated by the variation of the model prediction, increases first and then decreases with the order of the polynomial fit. Indeed, fidelity and robustness are two conflicting objectives in the development of data-driven empirical models. In consideration of both model fidelity and model robustness, a lower-order polynomial fit might outperform a higher-order polynomial fit.