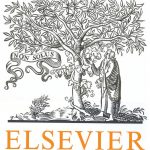
ترجمه مقاله نقش ضروری ارتباطات 6G با چشم انداز صنعت 4.0
- مبلغ: ۸۶,۰۰۰ تومان
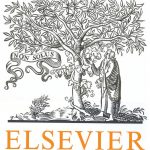
ترجمه مقاله پایداری توسعه شهری، تعدیل ساختار صنعتی و کارایی کاربری زمین
- مبلغ: ۹۱,۰۰۰ تومان
Abstract
With the innovation in 3D modeling software, more and more 3D models are becoming available in recent decades. To facilitate efficient retrieval and search of large 3D model databases, an effective shape classification algorithm is badly in need. In this paper, we propose a new feature descriptor named Principal Thickness Images (PTI) that encodes the boundary surface and the voxelized constituents of a 3D shape into three gray-scale images. With the support of PTI, we extend the kernel sparse representation-based classification from 2D case to non-rigid 3D models. Our classification algorithm inherits the robustness of kernel sparse representation and is able to achieve a high success rate and strong reliability on non-rigid models from the SHREC’11 non-rigid 3D models dataset. Numerous tests demonstrate superior performance of the proposed method over previous 3D shape classification approaches.
6. Conclusion
We proposed a novel descriptor called PTI. By encoding the thickness information of input 3D models, the PTI is not only invariant to the basic geometric transformation, such as translation, rotation, and scaling, but also insensitive to quasiisometric deformations, e.g., human body poses. Benefitting from partial volumetric information, the PTI is robust to small geometric noise added to the vertices of 3D models. To apply the PTI to 3D model classification, we combined the PTI with KSRC and presented a new 3D model classification algorithm. Extensive experimental results demonstrate the high accuracy, robustness, and effectiveness of our algorithm. However, we found that the MDS technique generally introduces shape distortion especially at extremity points although it basically eliminates pose differences. We will study if it is possible to obtain a better MDS method without shape distortion.