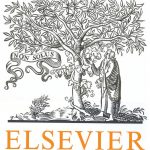
ترجمه مقاله نقش ضروری ارتباطات 6G با چشم انداز صنعت 4.0
- مبلغ: ۸۶,۰۰۰ تومان
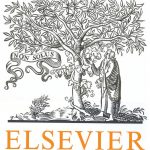
ترجمه مقاله پایداری توسعه شهری، تعدیل ساختار صنعتی و کارایی کاربری زمین
- مبلغ: ۹۱,۰۰۰ تومان
ABSTRACT
In classification, every feature of the data set is an important contributor towards prediction accuracy and affects the model building cost. To extract the priority features for prediction, a suitable feature selector is schemed. This paper proposes a novel memetic based feature selection model named Shapely Value Embedded Genetic Algorithm (SVEGA). The relevance of each feature towards prediction is measured by assembling genetic algorithms with shapely value measures retrieved from SVEGA. The obtained results are then evaluated using Support Vector Machine (SVM) with different kernel configurations on 11 + 11 benchmark datasets (both binary class and multi class). Eventually, a contrasting analysis is done between SVEGA-SVM and other existing feature selection models. The experimental results with the proposed setup provides robust outcome; hence proving it to be an efficient approach for discovering knowledge via feature selection with improved classification accuracy compared to conventional methods.
5. Discussion and conclusion
The experiments conducted in the study successfully demonstrate the beneficial factors of SVM-SVEGA over a range of other feature selection algorithms and prove it to be a promising technique. The results also show that the choices of the kernel function and feature selection technique have a profound effect on the performance of SVM for both binary and multi data classification. The effect of various SVM kernels impacted by the proposed SVEGA scheme is also investigated. Depending on the nature of the problem – binary class or multi class, appropriate kernels may be chosen. The study conducted can be comprehendingly substituted as a complete expert system modelfor deducing faster clinical diagnosis with better accuracy. The future work can test the proposed techniques on datasets from other domains. Further Ant Colony Optimization and Particle Swarm Analysis may be employed when low cost study is required.