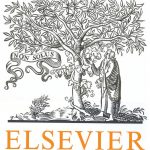
ترجمه مقاله نقش ضروری ارتباطات 6G با چشم انداز صنعت 4.0
- مبلغ: ۸۶,۰۰۰ تومان
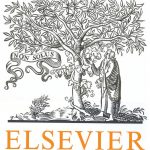
ترجمه مقاله پایداری توسعه شهری، تعدیل ساختار صنعتی و کارایی کاربری زمین
- مبلغ: ۹۱,۰۰۰ تومان
Abstract
Malware is a major threat to security and privacy of network users. A large variety of malware is typically spread over the Internet, hiding in benign traffic. New types of malware appear every day, challenging both the research community and security companies to improve malware identification techniques. In this paper we present MAGMA, MultilAyer Graphs for MAlware detection, a novel malware behavioral classifier. Our system is based on a Big Data methodology, driven by real-world data obtained from traffic traces collected in an operational network. The methodology we propose automatically extracts patterns related to a specific input event, i.e., a seed, from the enormous amount of events the network carries. By correlating such activities over (i) time, (ii) space, and (iii) network protocols, we build a Network Connectivity Graph that captures the overall “network behavior” of the seed. We next extract features from the Connectivity Graph and design a supervised classifier. We run MAGMA on a large dataset collected from a commercial Internet Provider where 20,000 Internet users generated more than 330 million events. Only 42,000 are flagged as malicious by a commercial IDS, which we consider as an oracle. Using this dataset, we experimentally evaluate MAGMA accuracy and robustness to parameter settings. Results indicate that MAGMA reaches 95% accuracy, with limited false positives. Furthermore, MAGMA proves able to identify suspicious network events that the IDS ignored.
9. Conclusions
We presented MAGMA, a classifier for malicious network activity identification. It leverages simple events collected from the network vantage point, where both the spatial and temporal recurrences of events allow MAGMA to capture a detailed picture of the activity involved in a malicious or benign activity using Big Data approaches. MAGMA models this by means of Network Connectivity Graphs, in which multiple graphs model the common events found by separately analyzing different protocols, and then fusing them in a single graph. A decision tree classifier is trained on a dataset where malicious and benign graphs are labeled by an oracle, which exposed a very heterogeneous set of malicious and benign activities. MAGMA thus results in a general purpose malware classifier, able to leverage common features that characterize several different families and variations of malware. We presented a performance evaluation using a real traffic trace obtained from a large ISP. MAGMA accuracy is over 95%, and its performance shows little sensitivity to parameter settings. MAGMA model is based on the extraction of recurrent events from the traffic surrounding a given seed. We acknowledge that MAGMA applicability is limited to only those threat families that exhibit recurrent patterns over time and over multiple hosts. MAGMA is intended to facilitate the identification of previously unknown malware and to support the forensic activity of a security analyst. We have shown that the MAGMA Network Connectivity Graph provides a rich and interpretable characterization of the malicious activity