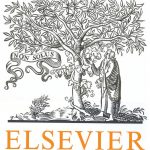
ترجمه مقاله نقش ضروری ارتباطات 6G با چشم انداز صنعت 4.0
- مبلغ: ۸۶,۰۰۰ تومان
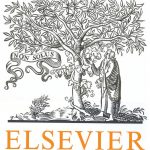
ترجمه مقاله پایداری توسعه شهری، تعدیل ساختار صنعتی و کارایی کاربری زمین
- مبلغ: ۹۱,۰۰۰ تومان
SUMMARY
Cancer progression involves the gradual loss of a differentiated phenotype and acquisition of progenitor and stem-cell-like features. Here, we provide novel stemness indices for assessing the degree of oncogenic dedifferentiation. We used an innovative one-class logistic regression (OCLR) machinelearning algorithm to extract transcriptomic and epigenetic feature sets derived from non-transformed pluripotent stem cells and their differentiated progeny. Using OCLR, we were able to identify previously undiscovered biological mechanisms associated with the dedifferentiated oncogenic state. Analyses of the tumor microenvironment revealed unanticipated correlation of cancer stemness with immune checkpoint expression and infiltrating immune cells. We found that the dedifferentiated oncogenic phenotype was generally most prominent in metastatic tumors. Application of our stemness indices to single-cell data revealed patterns of intra-tumor molecular heterogeneity. Finally, the indices allowed for the identification of novel targets and possible targeted therapies aimed at tumor differentiation.
Compounds Targeting with Cancer Stemness
To determine which target drugs might be useful against cancer stem cells, we used the Broad Institute’s Connectivity Map build 02 (CM) (Lamb et al., 2006), a public online tool (with registration) that allows users to predict compounds that can activate or inhibit based on a gene expression signature.
To further investigate about mechanism of actions (MoA) and drug-target we performed specific analysis within Connectivity Map tools (https://clue.io/) (Subramanian et al., 2017).
Using Connectivity Map (Query) in May 2017 having data available from a collection of cell lines (MCF, PC3, HL60 and SKMEL5) and 164 compounds as small molecules perturbagens. We obtained 33 mRNA expression signatures (one for each cancer type) by applying a differential expression analysis to samples with high mRNAsi and low mRNAsi, using the function TCGAanalyze_DEA from the the R/Bioconductor package TCGAbiolinks version 2.5.9 (Colaprico et al., 2016), carrying edgeR pipeline. The table with differentially expressed genes is reported as Table S3. Due to a limitation of the Connectivity Map tool that matches gene symbol and HG-U133A probe set (eg 200800_s_at) GPL96 platform ID, we had to remove duplicate IDs after sorting by decreasing jlogFCj. We selected the top 1000 genes (500 upregulated and 500 downregulated) where the number of differentially expressed genes was enough or considering the aggregation of upregulated or downregulated genes.
Connectivity MAP is a method similar to GSEA analysis and follows a 4 step approach: (i) looking for similarity between a query signature (diff.expr. genes) and expression profiles present in the dataset using pattern-matching strategy based on KolmogorovSmirnov test (ii) rank-ordering the list of genes according their diff.expr. relative to the control from the above expression profiles with significantly similarity (iii) comparison of each rank-ordered list with a query signature to specify when upregulated query genes appear in the proximity of the top of the list or near the bottom (‘‘positive connectivity’’) or vice versa (‘‘negative connectivity’’) producing an Enrichment Score (ES) from 1 to 1.