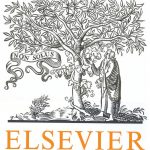
ترجمه مقاله نقش ضروری ارتباطات 6G با چشم انداز صنعت 4.0
- مبلغ: ۸۶,۰۰۰ تومان
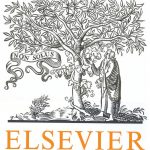
ترجمه مقاله پایداری توسعه شهری، تعدیل ساختار صنعتی و کارایی کاربری زمین
- مبلغ: ۹۱,۰۰۰ تومان
Abstract
This paper introduces a kernel-based sampling approach for image reconstruction and meshing. Given an input image and a user-specified number of points, the proposed method can automatically generate adaptive distribution of samples globally. We formulate the problem as an optimization to reconstruct the image by summing a small number of Gaussian kernels to approximate the given target image intensity or density. Each Gaussian kernel has the fixed size and the same energy. After the optimization, the samples are well distributed according to the image intensity or density variations as well as faithfully preserved the feature edges, which can be used to reconstruct high-quality images. Finally, we generate the adaptive triangular or tetrahedral meshes based on the well-spaced samples in 2D and 3D images. Our results are compared qualitatively and quantitatively with the state-of-the-art in image sampling and reconstruction on several examples by using the standard measurement criteria.
8. Conclusion
We have presented a new kernel-based adaptive sampling method, which is to optimize the positions of Gaussian kernels globally without explicit control of sampling population. The proposed method can be used in stipple drawing, image reconstruction and adaptive mesh generation in both 2D and 3D images. Our approach has not only improved the computational complexity in linear time O(n + m) compared with previous CVT-based methods, but also better captured the image features and reconstructed high-quality images, such as compared with CVT-based and Fattal’s methods. In the future, we will investigate the anisotropic sampling for image reconstruction and meshing, especially images with many sharp features. It is also possible to extend this framework to the surfaces. In order to further speed up the computation, the GPU-based parallel algorithm and implementation will be investigated.