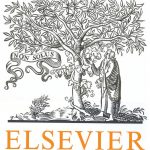
ترجمه مقاله نقش ضروری ارتباطات 6G با چشم انداز صنعت 4.0
- مبلغ: ۸۶,۰۰۰ تومان
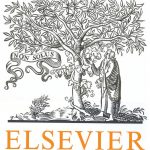
ترجمه مقاله پایداری توسعه شهری، تعدیل ساختار صنعتی و کارایی کاربری زمین
- مبلغ: ۹۱,۰۰۰ تومان
Abstract
Previous studies of bankruptcy prediction in imbalanced datasets analyze either the loss of prediction due to data imbalance issues or treatment methods for dealing with this issue. The current article presents a combined investigation of the degree of imbalance, loss of performance, and treatment methods. It determines which imbalanced class distributions jeopardize the performance of bankruptcy prediction methods and identifies the recovery capacities of treatment methods. The results show that an imbalanced distribution, in which the minority class represents 20%, significantly disturbs prediction performance. Furthermore, the support vector machine method is less sensitive than other prediction methods to imbalanced distributions, and sampling methods can recover a satisfactory portion of performance losses. Accordingly, this study provides a better understanding of the data imbalance issue in the field of corporate failure and serves as a methodological guide for designing bankruptcy prediction methods in imbalanced datasets.
5. Conclusion
We investigate the performance of bankruptcy prediction models in imbalanced datasets by analyzing three key notions: degree of imbalance, loss of performance, and sampling techniques. We establish which imbalanced distribution significantly damages prediction performance. Models built on training sets, in which bankrupt firms represent equal to or less than 20% of the total samples, suffer significantly diminished prediction performance. Although the performance of all classifiers is affected by imbalanced datasets, especially as that imbalance grows greater, the results that the SMV method is less sensitive. That is, it only suffers significant losses in performance in the most extreme scenarios (90/10 and 95/5 class proportions).
We also provide experimental results with regard to treatment methods and sampling techniques in imbalanced datasets. When we analyze the capacities of sampling techniques to recover prediction performance by balancing training sets, the results indicate an acceptable average recovery of 43.9%. Moreover, bankruptcy prediction models perform differently, depending on the sampling techniques used. In this regard, oversampling is a better choice, because it is most suitable for all type of prediction models and different training set sizes.
We also take a novel perspective that investigates the intercorrelations among the degree of data imbalance, the bankruptcy models’ loss of performance, and sampling techniques. We thereby fill a significant knowledge gap and make two main contributions -one methodological and one empirical- to bankruptcy prediction literature.