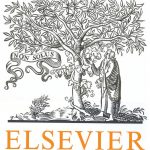
ترجمه مقاله نقش ضروری ارتباطات 6G با چشم انداز صنعت 4.0
- مبلغ: ۸۶,۰۰۰ تومان
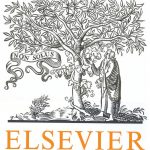
ترجمه مقاله پایداری توسعه شهری، تعدیل ساختار صنعتی و کارایی کاربری زمین
- مبلغ: ۹۱,۰۰۰ تومان
Abstract
Likely system invariants model properties that hold in operating conditions of a computing system. Invariants may be mined offline from training datasets, or inferred during execution. Scientific work has shown that invariants’ mining techniques support several activities, including capacity planning and detection of failures, anomalies and violations of Service Level Agreements. However their practical application by operation engineers is still a challenge. We aim to fill this gap through an empirical analysis of three major techniques for mining invariants in cloud-based utility computing systems: clustering, association rules, and decision list. The experiments use independent datasets from real-world systems: a Google cluster, whose traces are publicly available, and a Software-as-a-Service platform used by various companies worldwide. We assess the techniques in two invariants’ applications, namely executions characterization and anomaly detection, using the metrics of coverage, recall and precision. A sensitivity analysis is performed. Experimental results allow inferring practical usage implications, showing that relatively few invariants characterize the majority of operating conditions, that precision and recall may drop significantly when trying to achieve a large coverage, and that techniques exhibit similar precision, though the supervised one a higher recall. Finally, we propose a general heuristic for selecting likely invariants from a dataset.
9 CONCLUSIONS L IKELY SYSTEM INVARIANTS
can be mined for a variety of service computing systems, including cloud systems, web service infrastructures, datacenters, enterprise systems, IT services and utility computing systems, network services, distributed systems. They represent operational abstractions of normal system dynamics. The identification and the analysis of their violations support a range of operational activities, such as runtime anomaly detection, post mortem troubleshooting, capacity planning. In this work we have used two real-world datasets - the publicly available Google datacenter dataset and a dataset of a commercial SaaS utility computing platform - for assessing and comparing three techniques for invariant mining. Analysis and comparison was based on the common metrics coverage, recall and precision. The results provide insights into advantages and limitations of each technique, and practical suggestions to practitioners to establish the configuration of the mining algorithms and to select the number of invariants. The high-level findings are the following. A relatively small number of invariants allows to reach a relatively high coverage, i.e. they characterize the majority of executions. A small increase of the coverage of correct executions may produce a significant drop of recall and precision. The techniques exhibit similar precision, but the decision list supervised technique outperforms the unsupervised ones in recall. Finally, we presented a general heuristic for selecting a set of likely invariants from a dataset. All these results aim to fill the gap between past scientific studies and the concrete usage of likely system invariants by operations engineers.