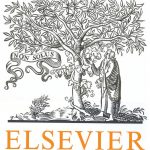
ترجمه مقاله نقش ضروری ارتباطات 6G با چشم انداز صنعت 4.0
- مبلغ: ۸۶,۰۰۰ تومان
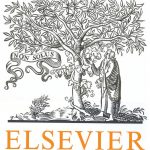
ترجمه مقاله پایداری توسعه شهری، تعدیل ساختار صنعتی و کارایی کاربری زمین
- مبلغ: ۹۱,۰۰۰ تومان
Abstract
The excellent performance of deep neural networks has enabled us to solve several automatization problems, opening an era of autonomous devices. However, current deep net architectures are heavy with millions of parameters and require billions of floating point operations. Several works have been developed to compress a pre-trained deep network to reduce memory footprint and, possibly, computation. Instead of compressing a pre-trained network, in this work, we propose a generic neural network layer structure employing multilinear projection as the primary feature extractor. The proposed architecture requires several times less memory as compared to the traditional Convolutional Neural Networks (CNN), while inherits the similar design principles of a CNN. In addition, the proposed architecture is equipped with two computation schemes that enable computation reduction or scalability. Experimental results show the effectiveness of our compact projection that outperforms traditional CNN, while requiring far fewer parameters.
5. Conclusions
In this paper, we proposed a multilinear mapping to replace the conventional convolution filter in Convolutional Neural Networks. The resulting structure’s complexity can be flexibly controlled by adjusting the number of projections in each mode through a hyper-parameter R. The proposed mapping comes with two computation schemes which either allow memory and computation reduction when R is small, or the scalability when R is large. Numerical results showed that with far fewer parameters, architectures employing our mapping could outperform standard CNNs. This are promising results and opens future research directions focusing on optimizing parameter R on individual convolution layers to achieve the most compact structure and performance.