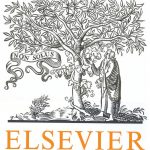
ترجمه مقاله نقش ضروری ارتباطات 6G با چشم انداز صنعت 4.0
- مبلغ: ۸۶,۰۰۰ تومان
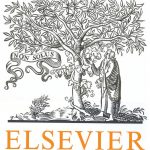
ترجمه مقاله پایداری توسعه شهری، تعدیل ساختار صنعتی و کارایی کاربری زمین
- مبلغ: ۹۱,۰۰۰ تومان
Background
Gastrostomy placement after intracerebral hemorrhage indicates the need for continued medical care and predicts patient dependence. Our objective was to determine the optimal machine learning technique to predict gastrostomy. Methods: We included 531 patients in a derivation cohort and 189 patients from another institution for testing. We derived and tested predictions of the likelihood of gastrostomy placement with logistic regression using the GRAVo score (composed of Glasgow Coma Scale 12, age >50 years, black race, and hematoma volume >30 mL), compared to other machine learning techniques (kth nearest neighbor, support vector machines, random forests, extreme gradient boosting, gradient boosting machine, stacking). Receiver Operating Curves (Area Under the Curve, [AUC]) between logistic regression (the technique used in GRAVo score development) and other machine learning techniques were compared. Another institution provided an external test data set. Results: In the external test data set, logistic regression using the GRAVo score components predicted gastrostomy (P < 0.001), however, with a lower AUC (0.66) than kth nearest neighbors (AUC 0.73), random forests (AUC 0.74), Gradient boosting machine (AUC 0.77), extreme gradient boosting (AUC 0.77), (P < 0.01 for all compared to logistic regression). Results from the internal test set were similar. Conclusions: Machine learning techniques other than logistic regression (eg, random forests, extreme gradient boost, and kth nearest neighbors) were significantly more accurate for predicting gastrostomy using the same independent variables. Machine learning techniques may assist clinicians in identifying patients likely to need interventions.
Summary
We found that machine learning techniques had higher accuracy in predicting gastrostomy after ICH compared to a validated ordinal prediction score using regression, even when using the same score components as independent variables. Machine learning techniques are likely to be useful to predict outcomes that have heretofore been predicted by logistic regression using ordinal scores. Machine learning may be broadly applicable for predicting complications and outcomes, given the ubiquitous need for accurate predictions and risk assessments in clinical medicine.