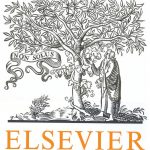
ترجمه مقاله نقش ضروری ارتباطات 6G با چشم انداز صنعت 4.0
- مبلغ: ۸۶,۰۰۰ تومان
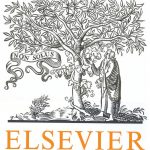
ترجمه مقاله پایداری توسعه شهری، تعدیل ساختار صنعتی و کارایی کاربری زمین
- مبلغ: ۹۱,۰۰۰ تومان
Abstract
Hyperspectral image (HSI) anomaly targets detection has been widely used in disaster alarm and military applications. Deep learning based HSI anomaly detector (AD) performs better by learning high-level features. However, the issues from heavy training computational burden and the model mismatch bring new challenges for online applications in the aspect of processing speed and detection accuracy. In this paper, an online Maximum-Distance-Pixel-Library(MDPL) method is proposed by using the most effective pixels to update deep autoencoder based HSI AD with less extra computation. Experimental results on two recorded hyperspectral images show that the proposed method outperforms the traditional real-time local Reed-Xiaoli based detector in term of accuracy and processing time. Compared with fully updating deep learning based HSI AD, the proposed method performs higher time efficiency without accuracy loss.
V. CONCLUSION
Online HSI AD is widely required in real applications. For deep learning based HSI AD which performs well in the offline mission, it is still a big challenge to overcome the model mismatch problem with less computational requirements in online application. In this paper, an online MDPL AD is proposed with less updating pixels by a maximum average distance strategy without accuracy loss. Experimental results on two real HSI datasets which have been embedded with anomaly targets show the proposed method reach up to 3.2 to 9.2 times speedup comparing to BLRXD and full updating SAE HSI AD respectively. It also gets better detection accuracy than the aformentioned comparison methods. In the future, a more efficient re-training pixel selection method needs to be further studied to overcome the problem that the size of MDPL may increase along with detection time.