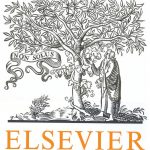
ترجمه مقاله نقش ضروری ارتباطات 6G با چشم انداز صنعت 4.0
- مبلغ: ۸۶,۰۰۰ تومان
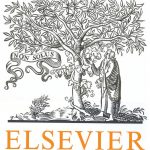
ترجمه مقاله پایداری توسعه شهری، تعدیل ساختار صنعتی و کارایی کاربری زمین
- مبلغ: ۹۱,۰۰۰ تومان
Adverse
drug events (ADEs) contribute significantly to morbidity and mortality in the healthcare system. The availability of digitalised hospitals’ narrative clinical data offers a potentially rich resource to enhance pharmacovigilance efforts to manage potential safety issues arising from real-world use of drugs. The goal of this paper was to establish a foundation for creating an evaluation corpus by developing a set of annotation guidelines to achieve high inter-annotator agreement (IAA) and to evaluate the performance of basic entity identification tools for drugs, adverse events (AEs) and drug-AE relationships from 100 discharge summaries of a tertiary hospital in Singapore. Two teams of three annotators worked independently on text annotation using Knowtator. Three-way IAA of 86%, 70% and 49% were achieved for drugs, AEs and drug-AE relationships respectively. The performance of the machine algorithm was evaluated against annotations made by at least two annotators, with a recall of 84% and precision of 73% for drugs and a recall of 67% and precision of 53% for AEs. The high recall and precision for drug entity extraction suggests that machine pre-annotation of drugs followed by human annotation of AEs and drug-AE relationships could be a feasible approach in expediting the process of creating a larger evaluation corpus. Non-matches between machine and human annotations were examined to identify ways to further refine the algorithm. When successfully implemented, the identification of ADEs could greatly support pharmacovigilance work in characterising the magnitude and scope of ADEs and prioritising interventions to improve the drug safety.
5. Future research
directions Classical natural language processing models are trained on well written text. Accuracy of tagging drops significantly with unstructured text, abundant abbreviations, misspellings and ungrammatical constructs. While our study showed the feasibility of our methods, more work is needed to establish a drug safety surveillance mechanism. Our plan for the future is to work on improved text mining algorithms for entity and relationship extraction.1. Improve inter-annotator agreement by refining the guidelines on annotation. 2. Develop an evaluation corpus to benchmark the performance of the text mining algorithms. 3. Expand the reference gazetteer by extracting AE terms from approved drug labels and other medical abbreviation lists. 4. Investigate deep machine learning strategies, such as those recently described in the review articles [23–25], to iteratively improve recognition of patterns that are likely to be ADEs. 5. Evaluate algorithm performance on discharge summaries from other hospitals that have different EMR technology platforms and clinician practices for writing discharge summaries to assess the applicability of the algorithms in the broader health care system. 6. Explore text-mining strategies, such as frequent itemset mining, that would be suited for discovery of previously unknown drug-AE relationships.