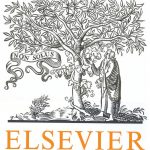
ترجمه مقاله نقش ضروری ارتباطات 6G با چشم انداز صنعت 4.0
- مبلغ: ۸۶,۰۰۰ تومان
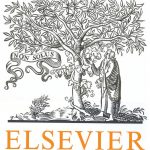
ترجمه مقاله پایداری توسعه شهری، تعدیل ساختار صنعتی و کارایی کاربری زمین
- مبلغ: ۹۱,۰۰۰ تومان
Abstract
In this article, we introduce a new hybrid model called hesitant N-soft sets by a suitable combination of hesitancy with N-soft sets, a model that extends N-soft sets. Our novel concept is illustrated with real life examples. Moreover, we investigate some useful properties of hesitant N-soft sets and construct fundamental operations on them. We describe potential applications of hesitant N-soft sets in group decision-making, and finally we present some group decision-making methods as algorithms.
4 Conclusion
N-soft sets are the extended and applicable version of soft sets that can deal with both binary and non-binary evaluations. That model is widely used to make decisions in general real situations, and examples were provided in the founding reference Fatimah et al. (2018a). An interesting feature of its design is that contrary to the case of soft sets, the introduction of multinary categorizations is naturally compatible with hesitation. However the model by N-soft sets is unable to make decisions when data collection produces hesitancy. In this research article we have introduced a new extended model of N-soft sets as an answer for this drawback, which is the natural hybridization of N-soft sets and hesitancy. In addition, the case N = 2 reduces to incomplete soft sets. Hesitant data may come from sources like hesitation on the side of the decision-maker, or the combination of the evaluations provided by various decision makers. The fundamental concept that we introduced is called hesitant N-soft set or simply HNSS. We have put forward real life examples that adopt the format of HNSSs. A related concept is hesitant N-tuple, which appears as an inherent element of the practical description of HNSSs. We have introduced scores of hesitant N-tuples in order to have new tools for prioritization. Particularly, scores are adequate for implementation of GDM algorithms based on HNSSs. We have set forth an algorithm for making decisions that can account for parameters with unequal weights. Moreover, we have investigated the properties and basic operations of HNSSs, as well as their interaction with less flexible models. In the future, we expect to extend our research work on related hybrid models with the construction of (1) N-soft mF rough graphs, (2) N-soft rough mF graphs, (3) Hesitant Nsoft graphs, (4) Hesitant N-soft hypergraphs, and (5) Hesitant Pythagorean fuzzy graphs