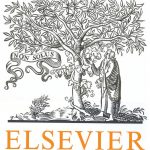
ترجمه مقاله نقش ضروری ارتباطات 6G با چشم انداز صنعت 4.0
- مبلغ: ۸۶,۰۰۰ تومان
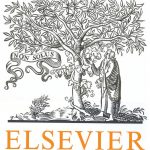
ترجمه مقاله پایداری توسعه شهری، تعدیل ساختار صنعتی و کارایی کاربری زمین
- مبلغ: ۹۱,۰۰۰ تومان
abstract
In this paper, Gaussian process regression (GPR) for fingerprinting based localization is presented. In contrast to general regression techniques, the GPR not only infers the posterior received signal strength (RSS) mean but also the variance at each fingerprint location. The GPR does take into account the variance of input i.e., noisy RSS data. The hyper-parameters of GPR are estimated using trust-region-reflective algorithm. The Cramér-Rao bound is analysed to highlight the performance of the parameter estimator. The posterior mean and variance of RSS data is utilized in fingerprinting based localization. The principal component analysis is employed to choose the k strongest wi-fi access points (APs). The performance of the proposed algorithm is validated using using real field deployments. Accuracy improvements of 10% and 30% are observed in two sites compared to the Horus fingerprinting approach.
5. Conclusion and Future Work
In this paper, localization accuracy with respect to the standard Horus technique is achieved at the expense of O(N3) computational complexity. This complexity arises from wi-fi signal prediction at each location using Gaussian process regression (GPR). To further reduce it, network segmentation having complexity O(N3), N ≤ N, can be employed. Future work includes developing a real-time positioning system that localize as we go, i.e, calibration-free localization. We collect approximately ten wi-fi signal snap-shots at each location to minimize the effect of small-scale fading. The performance of this localization system will be affected if we collect a very few number of snap-shots. This is because wi-fi signal is not very reliable. In this case, at some locations, corresponding wi-fi signal may be treated as outliers. If the number of such outliers is less than the 50% of the total wi-fi observations at all locations, Gaussian process with Student-t likelihood can be utilized, in this context to deal with outliers. Although, conditional posterior is intractable for this non-Gaussian likelihood, and approximation is required with Markov chain Monte Carlo, Laplace approximation, or expectation propagation algorithm