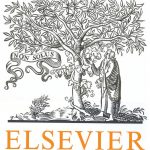
ترجمه مقاله نقش ضروری ارتباطات 6G با چشم انداز صنعت 4.0
- مبلغ: ۸۶,۰۰۰ تومان
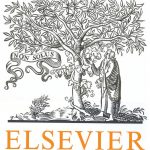
ترجمه مقاله پایداری توسعه شهری، تعدیل ساختار صنعتی و کارایی کاربری زمین
- مبلغ: ۹۱,۰۰۰ تومان
abstract
The stock price prediction is an important issue in stock markets because it will result in significant benefits and impacts for investor. In contrast to traditional time series, fuzzy time series can solve the forecast problem with historical data of linguistic values. In order to improve forecast performance of fuzzy timeseries models, this study replaced fuzzy logical relationships with rule-based algorithm to extract forecast rules from time-series observations. Therefore, this paper developed a novel fuzzy time-series model based on rough set rule induction for forecasting stock index, and this study has four contributions to improve forecast accuracy and provide investment point (in right time) to investors: (1) Proposed a novel fuzzy time-series model to improve forecast accuracy, (2) rough sets are employed to generate forecasting rules to replace fuzzy logical relationship rules based on the lag period, (3) utilized adaptive expectation model to strengthen forecasting performance, and based on the meaning of adaptive parameter to observe stock fluctuation and oscillation, and (4) proposed buy and sell rules to calculate the profit and based on three different scenarios to provide investment suggestion to investor as references. For evaluating the proposed model, we practically collected TAIEX, Nikkei, and HSI stock price from 1998 to 2012 years as experimental dataset, and compared the listing models under three error indexes and profits criteria. The results show that the proposed method outperforms listing models in error indexes and profits.
5. Conclusion
This paper has proposed a novel fuzzy time-series model based on rough set rule induction for forecasting stock index, the proposed model utilized rough set LEM2 algorithm to generate forecast rules, it’s different from previous fuzzy time series using FLRs and employed adaptive expectation model to strengthen forecasting performance. The results have shown the proposed model with better forecast performance in accuracy and profit. From three stock markets data with 45 sub-datasets, we can conclude that there are four findings as follows.
(1) The number of linguistic interval Table 7 show that the nine linguistic intervals will result in the higher forecasting performance. From Yu [36], the more linguistic intervals utilize the more get forecast performance. However, based on Miller [10], the appropriate number of category for human shorten memory function is seven, or seven plus or minus two. That is, many linguistic intervals would disturb human shorten memory function for investors. Therefore, this study suggests that nine linguistic intervals with rule filter is a good approach.
(2) Whether rule filtered After filtering the generated rules (remove the “support” less than 2), the higher support rules can get the better forecasting performance as Table 7. i.e., the higher support rules in the training data have higher data matching, and then the forecast performance of proposed model will be better for testing data.