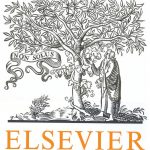
ترجمه مقاله نقش ضروری ارتباطات 6G با چشم انداز صنعت 4.0
- مبلغ: ۸۶,۰۰۰ تومان
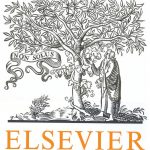
ترجمه مقاله پایداری توسعه شهری، تعدیل ساختار صنعتی و کارایی کاربری زمین
- مبلغ: ۹۱,۰۰۰ تومان
Abstract
The efficiency of the probabilistic neural network (PNN) is very sensitive to the cardinality of a considered input data set. It results from the design of the network’s pattern layer. In this layer, the neurons perform an activation on all input records. This makes the PNN architecture complex, especially for big data classification tasks. In this paper, a new algorithm for the structure reduction of the PNN is put forward. The solution relies on performing a fuzzy c–means data clustering and selecting PNN’s pattern neurons on the basis of the obtained centroids. Then, to activate the pattern neurons, the algorithm chooses input vectors for which the highest values of the membership coefficients are determined. The proposed approach is applied to the classification tasks of repository data sets. PNN is trained by three different classification procedures: conjugate gradients, reinforcement learning and the plugin method. Two types of kernel estimators are used to activate the neurons of the network. A 10–fold cross validation errors for the original and the reduced PNNs are compared. Received results confirm the validity of the introduced algorithm.
Conclusions
In this paper, a new algorithm for the reduction of a PNN’s pattern layer was proposed. It relied on computing the centroids from the original input data by means of the FCM algorithm. The new set of the pattern neurons was established based on the input vectors that were nearest to the centroids in terms of a fuzzy similarity. Three different values of the m fuzzifier were chosen. Various PNN training procedures were selected for evaluation purposes, in particular: conjugate gradients, reinforcement learning and the plugin method. The algorithm was tested on six repository data classification tasks by collating the full structure PNN classification error, the minimum centroids-based error and the final reduction error, all obtained by means of a 10-fold cross validation procedure; the PNN reduction ratio and the decreases in the classification error after reduction were also computed. Furthermore, in order to make the comparison more comprehensive, a two sample t-test was performed to confirm the statistical significance of the results, the computational time of the algorithm was indicated and a comparison to the existing approaches was performed. As shown, the algorithm provided satisfactory outcomes in all the evaluated factors, making it useful in reduction tasks in general.