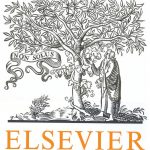
ترجمه مقاله نقش ضروری ارتباطات 6G با چشم انداز صنعت 4.0
- مبلغ: ۸۶,۰۰۰ تومان
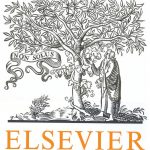
ترجمه مقاله پایداری توسعه شهری، تعدیل ساختار صنعتی و کارایی کاربری زمین
- مبلغ: ۹۱,۰۰۰ تومان
Abstract
In online shopping, product returns are very common. Liberal return policies have been widely used to attract shoppers. However, returns often increase expense and inconvenience for all parties involved: customers, retailers, and manufacturers. Despite the large fraction of purchases that are returned, there are few systematic studies to explain the underlying forces that drive return requests, and to assess the return propensity at the level of individual purchases (i.e., a particular customer purchasing a particular product), rather than in aggregate. To this end, in this paper, we provide a systematic framework for personalized predictions of return propensity. These predictions can help retailers enhance inventory management, improve customer relationships, and reduce return fraud and abuse. Specifically, we treat product returns as a result of inconsistency arising during a commercial transaction. We decompose this inconsistency into two components, one for the buying phase (e.g., product does not match description) and another for the shipping phase (e.g., product damaged during shipping). Along these lines, we introduce a generalized return propensity latent model (RPLM). We further propose a complete framework, called fused return propensity latent model (FRPLM), to jointly model the correlation among user profiles, product features, and return propensity. We present comprehensive experimental results with real-world data to demonstrate the effectiveness of the proposed method for assessing return propensity.
7. Conclusions
In this paper, we investigated what are the underlying forces that drive product return requests, and how to assess return propensity of specific customers for specific products. Specifically, we assumed that product returns are due to the inconsistency between expected and received product. To model this inconsistency, we devised a generalized return propensity latent model (RPLM) framework to model the inconsistency between expected product and shipped product, and the inconsistency between shipped product and received product, which arises during the buying and shipping phases, respectively. Furthermore, we proposed an enhanced specification, called fused return propensity latent model (FRPLM), to jointly model the correlation among user profiles, product features, and return propensity. Finally, we conducted extensive experiments on real-world data from an online shopping site, which contain orders, returns, and refunds, as well as side information, including as user profiles and product features. As demonstrated by the experimental results, a view of inconsistency generated along with retailer–customer exchange process can better assess return propensity, with interpretable explanations. The performance improvement of our proposed method is substantial, compared to benchmark methods.