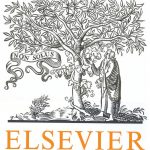
ترجمه مقاله نقش ضروری ارتباطات 6G با چشم انداز صنعت 4.0
- مبلغ: ۸۶,۰۰۰ تومان
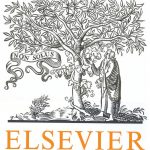
ترجمه مقاله پایداری توسعه شهری، تعدیل ساختار صنعتی و کارایی کاربری زمین
- مبلغ: ۹۱,۰۰۰ تومان
Abstract
To address the sequential changes of images including poses, in this paper we propose a recurrent regression neural network (RRNN) framework to unify two classic tasks of cross-pose face recognition on still images and videos. To imitate the changes of images, we explicitly construct the potential dependencies of sequential images so as to regularizing the final learning model. By performing progressive transforms for sequentially adjacent images, RRNN can adaptively memorize and forget the information that benefits for the final classification. For face recognition of still images, given any one image with any one pose, we recurrently predict the images with its sequential poses to expect to capture some useful information of other poses. For video-based face recognition, the recurrent regression takes one entire sequence rather than one image as its input. We verify RRNN in still face image dataset MultiPIE and face video dataset YouTube Celebrities (YTC). The comprehensive experimental results demonstrate the effectiveness of the proposed RRNN method.
5. Conclusion
In this paper, we proposed a Recurrent Regression Neural Network (RRNN) to unify two classic face recognition tasks including cross-pose face recognition and video-based face recognition. In RRNN, three basic units are considered to model a potential sequence data. The first unit is the encoder-decoder, which is used to model sequential reconstruction. The second unit is to constrain the globality of the sequence. The final one is to utilize the discriminative label information. By properly choosing the configuration for different tasks, we can benefit from these units. Experimental results strongly indicate our RRNN achieves the best recognition results compared with those state-of-the art methods.