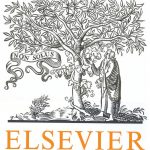
ترجمه مقاله نقش ضروری ارتباطات 6G با چشم انداز صنعت 4.0
- مبلغ: ۸۶,۰۰۰ تومان
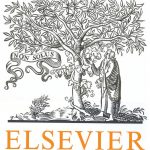
ترجمه مقاله پایداری توسعه شهری، تعدیل ساختار صنعتی و کارایی کاربری زمین
- مبلغ: ۹۱,۰۰۰ تومان
Abstract
With the remarkable success achieved by the Convolutional Neural Networks (CNNs) in object recognition recently, deep learning is being widely used in the computer vision community. Deep Metric Learning (DML), integrating deep learning with conventional metric learning, has set new records in many fields, especially in classification task. In this paper, we propose a replicable DML method, called Include and Exclude (IE) loss, to force the distance between a sample and its designated class center away from the mean distance of this sample to other class centers with a large margin in the exponential feature projection space. With the supervision of IE loss, we can train CNNs to enhance the intra-class compactness and inter-class separability, leading to great improvements on several public datasets ranging from object recognition to face verification. We conduct a comparative study of our algorithm with several typical DML methods on three kinds of networks with different capacity. Extensive experiments on three object recognition datasets and two face recognition datasets demonstrate that IE loss is always superior to other mainstream DML methods and approach the state-of-the-art results.
5. Conclusion and future work
In this paper, we propose a powerful and replicable DML method, which enforces the mean inter-class distance larger than the intra-class distance with a margin, to enhance the discriminability of the deeply learned features in object recognition and face verification. Extensive experiments on several public datasets have convincingly demonstrated the effectiveness of our method. The results also exhibit the excellent generalization of IE loss in various size of CNNs. Instead of requiring a superior neighborhood sampling strategy, our approach only uses mini-batch based SGD to conduct the experiments, avoiding the exponentially increased computational complexity of image pairs or triplets. Maybe a better hard sample mining strategy could improve the performance further. Inspired by the outstanding performance of IE loss in object recognition and face recognition, we will explore its extension in the case where the swarm intelligent methods are exploited to optimize the clustering algorithm [57, 58] in the following work. In the future, we will delve into DML to explore its extensive applications to other tasks.