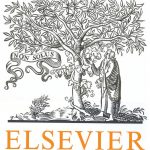
ترجمه مقاله نقش ضروری ارتباطات 6G با چشم انداز صنعت 4.0
- مبلغ: ۸۶,۰۰۰ تومان
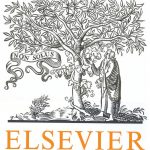
ترجمه مقاله پایداری توسعه شهری، تعدیل ساختار صنعتی و کارایی کاربری زمین
- مبلغ: ۹۱,۰۰۰ تومان
The ability of evolutionary algorithms (EAs) to manage a set of solutions, even attending multiple objectives, as well as their ability to optimize any kinds of values, allows them to fit very well some parts of the data-mining (DM) problems, whose native learning techniques usually associated with the inherent DM problem are not able to solve. Therefore, EAs are widely applied to complement or even replace the classical DM learning approaches. This application of EAs to the DM process is usually named evolutionary data mining (EDM). This contribution aims at showing a glimpse of the EDM field current state by focusing on the most cited papers published in the last 10 years. A descriptive analysis of the papers together with a bibliographic study is performed in order to differentiate past and current trends and to easily focus on significant further developments. Results show that, in the case of the most cited studied papers, the use of EAs on DM tasks is mainly focused on enhancing the classical learning techniques, thus completely replacing them only when it is directly motivated by the nature of problem. The bibliographic analysis is also showing that even though EAs were the main techniques used for EDM, the emergent evolutionary computation algorithms (swarm intelligence, etc.) are becoming nowadays the most cited and used ones. Based on all these facts, some potential further directions are also discussed.
7 | CONCLUSIONS
EDM is a prolific field that takes advantage from the application of EAs in order to enhance or to replace the typical learning algorithms associated with the different problems or DM techniques. EAs are powerful population-based optimization techniques that are able to improve or even learn any type of predictive or descriptive model, evolving their structure and/or definition parameters together. In this review article the most cited contributions on EDM from the last 10 years (including the current year) have been analyzed from two different points of views. First, analyzing the five most cited papers per year. Second, contrasting the classical EA-based approaches with the emergent-EC based ones by focusing on the EDM approaches that can be found within the 10% of the most cited papers from the ISI WoS per year. These top cited papers have been mainly found in the classification and regression areas, addressing a high number of particular applications, imbalanced classification problems, multiple objectives and interpretability issues, and TS forecasting. A number of contributions have been also found on unsupervised learning, addressing mainly clustering problems, and eventually performing AR mining and subgroup discovery.