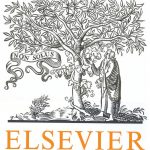
ترجمه مقاله نقش ضروری ارتباطات 6G با چشم انداز صنعت 4.0
- مبلغ: ۸۶,۰۰۰ تومان
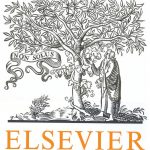
ترجمه مقاله پایداری توسعه شهری، تعدیل ساختار صنعتی و کارایی کاربری زمین
- مبلغ: ۹۱,۰۰۰ تومان
Abstract
For decomposable score-based structure learning of Bayesian networks, existing approaches first compute a collection of candidate parent sets for each variable and then optimize over this collection by choosing one parent set for each variable without creating directed cycles while maximizing the total score. We target the task of constructing the collection of candidate parent sets when the score of choice is the Bayesian Information Criterion (BIC). We provide new non-trivial results that can be used to prune the search space of candidate parent sets of each node. We analyze how these new results relate to previous ideas in the literature both theoretically and empirically. We show in experiments with UCI data sets that gains can be significant. Since the new pruning rules are easy to implement and have low computational costs, they can be promptly integrated into all state-of-the-art methods for structure learning of Bayesian networks.
Conclusions
This paper presents new non-trivial pruning rules to be used with the Bayesian Information Criterion (BIC) score for learning the structure of Bayesian networks. The derived theoretical bounds extend previous results in the literature and can be promptly integrated into existing solvers with minimal effort and computational costs. They imply faster computations without losing optimality. The very computationally efficient version of the new rules imply gains of around 20% with respect to previous work, according to our experiments, while the most computationally demanding pruning achieves around 50% more pruning than before. Pruning rules for other widely used scores such as the Bayesian Dirichlet equivalent uniform (BDeu) have been devised [13] and some researchers conjecture that they cannot be improved. Similarly, we conjecture that further bounds for the BIC score are unlikely to exist unless for some particular cases and situations. This can be studied in a future work, as well as means to devise smart strategies to tune the theorem parameters and improve their pruning capabilities.