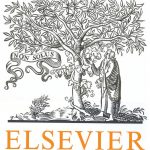
ترجمه مقاله نقش ضروری ارتباطات 6G با چشم انداز صنعت 4.0
- مبلغ: ۸۶,۰۰۰ تومان
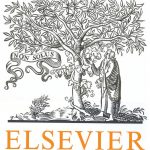
ترجمه مقاله پایداری توسعه شهری، تعدیل ساختار صنعتی و کارایی کاربری زمین
- مبلغ: ۹۱,۰۰۰ تومان
abstract
The unprecedented prevalence of ubiquitous sensing will revolutionise the Future Internet where stateof-the-art Internet-of-Things (IoT) is believed to play the pivotal role. In the fast forwarding IoT paradigm, hundreds of billions of things are estimated to be deployed which would give rise to an enormous amount of data. Cloud computing has been the prevailing choice for controlling the connected things and the data, and providing intelligence based on the data. But response time and network load are on the higher side for cloud based solutions. Recently, edge computing is gaining growing attention to overcome this by employing rule-based intelligence. However, requirements of rules do not scale well with the proliferation of things. At the same time, rules fail in uncertain events and only offer pre-assumed intelligence. To counter this, this paper proposes a novel idea of leveraging the belief-network with the edge computing to utilize as an IoT edge-controller the aim of which is to offer low-level intelligence for IoT applications. This low-level intelligence along with cloud-based intelligence form the distributed intelligence in the IoT realm. Furthermore, a learning approach similar to reinforcement learning has been proposed. The approach, i.e. enabling a Future IoT Controller (FITC) has been verified with a simulated SmartHome scenario which proves the feasibility of the low-level intelligence in terms of reducing rules domination, faster response time and prediction through learning experiences at the edge.
6. Conclusions
Internet of Things, the next technological revolution in the current world, applications have so far been relied on cloud-based solutions for computations, analytics, etc. While the advantages offered by cloud-based solutions cannot be disregarded; the need for computations, analytics closer to the things, i.e. at the edge is gaining growing attention recently. Edge analytics are dominated by rules which are computationally expensive and fail to scale well with the ever-mounting number of things in the IoT paradigm which mandate to look into alternative solution such as AI based solutions. AI based solutions can also cater for uncertain and new events. The paper has presented an AI based distributedintelligence assisted Future Internet of Things Controller (FITC) that utilizes both edge and cloud based intelligence. More specifically, edge controller is employed to provide low-level intelligence and cloud controller would provide high-level intelligence.
It is foreseen that the combination of IoT and AI is inseparable, and edge controller by utilizing AI techniques closer to the things would allow necessary edge analytics. Belief-network enables to make decisions based on prior-belief and it further can learn new belief based on probability. The proposed IoT edge controller further exploits capability similar to the reinforcement learning to learn experiences which can then be used for learning probability and making prediction. To the best of our knowledge, this paper is the first to propose such novel idea of employing belief-network and reinforcement learning at the edge of IoT. The feasibility of the proposed approach has been backed up by simulated SmartHome scenario on a raspberry pi as a proof-of-concept. Furthermore, mathematical formulas for obtaining probability, new belief and rules for belief-network have been presented in this paper. Results conveyed here suggest that employing beliefnetwork considerably reduces requirement of rules and enables faster response time for the edge controller; it also allows learning new belief based on experiences. Making prediction is one of the important capabilities that was missing from earlier edge solutions which this paper has successfully achieved to make prediction of the missing ConIn. The paper has also presented algorithms to achieve the aforementioned capabilities. Further, an algorithm to provide meaning to the raw-data inspired by the informationknowledge hierarchy has also been presented. Given the evidences reported in this paper, the approach can be used in many IoT applications such as SmartHome, SmartFarming, SmartHealth, Waste Management, etc. which can be explored in the future.