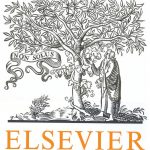
ترجمه مقاله نقش ضروری ارتباطات 6G با چشم انداز صنعت 4.0
- مبلغ: ۸۶,۰۰۰ تومان
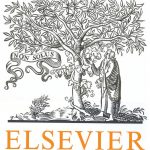
ترجمه مقاله پایداری توسعه شهری، تعدیل ساختار صنعتی و کارایی کاربری زمین
- مبلغ: ۹۱,۰۰۰ تومان
Frequent pattern mining generates a lot of candidates, which requires a lot of memory usage and mining time. In real applications, a small number of frequent patterns are used. Therefore, the mining of top-rank-k frequent patterns, which limits the number of mined frequent patterns by ranking them in frequency, has received increasing interest. This paper proposes the iNTK algorithm, which is an improved version of the NTK algorithm, for mining top-rank-k frequent patterns. This algorithm employs an N-list structure to represent patterns. The subsume concept is used to speed up the process of mining top-rank-k patterns. The experiments are conducted to evaluate iNTK and NTK in terms of mining time and memory usage for eight datasets. The experimental results show that iNTK is more efficient and faster than NTK.
1. Introduction
An expert system is an intelligent system that solves the complex problems based on knowledge throughout inference procedures. Generally, there are three components in an expert system including knowledge base, inference engine and user interface (Jackson, 1999). The central of expert systems is the knowledge base, because it contains the problem solving knowledge of the particular application (Ahmed, 2008). Therefore, the reduction of this knowledge space plays a big role in the implemented performance of expert systems. Association rules are important of the knowledge (Daniel & Viorel, 2004; Guil, Bosch, Túnez, & Marín, 2003) which represent the relationships between items in a dataset. To generate association rules, traditional approaches first mine frequent patterns which are itemsets, subsequences, and substructures that appear in large transactions or relational datasets with a frequency no less than a given threshold. After that, the system uses these frequent patterns and the minimum confidence to find all rules. Two above phrases require a lot of memory usage and mining time. Therefore, the reduction of time to mine frequent patterns is very useful to enhance expert systems.
6. Conclusion and future work
This paper presents an efficient improvement algorithm called iNTK to mine top-rank-k frequent patterns. The advantage of iNTK lies in that it uses N-list and subsume index of 1-patterns. N-list store information shorter than Node-list and subsume index help iNTK directly mining in case of patterns belonged to top-rank-k table contain other 1-patterns in their subsume set. This causes that iNTK consume less memory and runtime. Extensive experiments show that iNTK outperforms NTK for various datasets.
استخراج الگوهای متناوب کاندیدهای بسیاری تولید میکند که نیاز به صرف مقدار زیادی از حافظه و زمان استخراج دارد. در کاربردهای واقعی، از تعداد کمی از الگوهای متناوب استفاده می شود. بنابراین، استخراج K الگوی برتر متناوب که تعداد الگوهای متناوب استخراج شده را با رتبه بندی آنها برحسب تناوب محدود میکند، مورد توجه قرار گرفته است. این مقاله الگوریتم iNTK را پیشنهاد می کند که یک نسخه بهبود یافته از الگوریتم NTK برای استخراج K الگوی برتر متناوب است. این الگوریتم از یک ساختار N-لیست برای نشان دادن الگوها استفاده میکند. این مفهوم استنتاجی برای سرعت بخشیدن به روند استخراج K الگوی برتر مورد استفاده قرار میگیرد. آزمایش هایی برای ارزیابی iNTK و NTK برحسب زمان استخراج و مصرف حافظه برای هشت مجموعه داده انجام گرفته است. نتایج تجربی نشان می دهد که iNTK کارآمد تر و سریعتر از NTK است.
1. معرفی
سیستم خبره یک سیستم هوشمند است که مسائل پیچیده مبتنی بر دانش را از طریق روشهای استنتاج حل میکند. به طور کلی، سه مولفه در یک سیستم خبره وجود دارد از جمله پایگاه دانش، موتور استنتاج و رابط کاربری (Jackson ،1999). مرکز سیستم های خبره پایگاه دانش است، چراکه حاوی دانش حل مساله یک کاربرد خاص می باشد (Ahmed ، 2008). بنابراین، کاهش این فضای دانش نقش بزرگی در عملکرد اجرایی سیستم های خبره ایفا می کند. قوانین وابستگی دانش (Daniel و Viorel، 2004؛ Guil، Bosch ، Tunez ، و Marín ، 2003) که نشان دهنده روابط بین اقلام در یک مجموعه داده اند ، مهم هستند. برای ایجاد قوانین وابستگی، رویکردهای سنتی ابتدا الگوهای متناوب شامل مجموعه آیتم ها، زیردنباله ها و زیر ساختها را استخراج میکنند که در تراکنش های بزرگ و یا مجموعه داده های رابطه ای با تناوب کمتر از یک آستانه داده شده ظاهر می شوند. پس از آن، این سیستم از این الگوهای متناوب و حداقل اطمینان برای یافتن تمام قوانین استفاده میکند. دو عبارت بالا به مقدار زیادی حافظه و زمان استخراج نیاز دارد. بنابراین، کاهش زمان استخراج الگوهای متناوب به منظور بهبود سیستم های خبره بسیار مفید است.
6. نتیجه گیری و کارهای آینده
در این مقاله یک الگوریتم بهبودیافته کارآمد به نام iNTK برای استخراج K الگوی برتر متناوب ارائه شد. مزیت iNTK در استفاده از N-لیست و شاخص استنتاج 1-الگوها نهفته است. N-لیست نسبت به لیست-گره اطلاعات کمتری ذخیره کرده و شاخص استنتاج به طور مستقیم به iNTK در استخراج الگوهای متعلق به جدول K رتبه برتر حاوی 1-الگوها در مجموعه استنتاج کمک میکند. این باعث می شود که iNTK مصرف حافظه و زمان اجرای کمتری داشته باشد. آزمایش های گسترده نشان می دهد که iNTK از NTK برای مجموعه داده های مختلف بهتر عمل میکند.