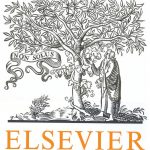
ترجمه مقاله نقش ضروری ارتباطات 6G با چشم انداز صنعت 4.0
- مبلغ: ۸۶,۰۰۰ تومان
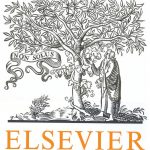
ترجمه مقاله پایداری توسعه شهری، تعدیل ساختار صنعتی و کارایی کاربری زمین
- مبلغ: ۹۱,۰۰۰ تومان
Abstract
Support vector machine (SVM) has been used widely for classification of electroencephalogram (EEG) signals for the diagnosis of neurological disorders such as epilepsy and sleep disorders. SVM shows good generalization performance for high dimensional data due to its convex optimization problem. The incorporation of prior knowledge about the data leads to a better optimized classifier. Different types of EEG signals provide information about the distribution of EEG data. To include prior information in the classification of EEG signals, we propose a novel machine learning approach based on universum support vector machine (USVM) for classification. In our approach, the universum data points are generated by selecting universum from the EEG dataset itself which are the interictal EEG signals. This removes the effect of outliers on the generation of universum data. Further, to reduce the computation time, we use our approach of universum selection with universum twin support vector machine (UTSVM) which has less computational cost in comparison to traditional SVM. For checking the validity of our proposed methods, we use various feature extraction techniques for different datasets consisting of healthy and seizure signals. Several numerical experiments are performed on the generated datasets and the results of our proposed approach are compared with other baseline methods. Our proposed USVM and proposed UTSVM show better generalization performance compared to SVM, USVM, Twin SVM (TWSVM) and UTSVM. The proposed UTSVM has achieved highest classification accuracy of 99 % for the healthy and seizure EEG signals.
Conclusions
On the basis of the experimental results, it can be stated that our universum based approach gives better generalization performance for the classification of EEG signals as compared to the existing approaches. Our method of selection of universum points has proved to be a promising approach for the classification of healthy and seizure EEG signals. Also, the effect of outliers on the universum is reduced by using the universum from the EEG dataset itself i.e., the seizure free EEG signal. The distribution of interictal (seizure free) signals provides prior information about the distribution of healthy and seizure signals and also lies in between the two classes. Based on the experimental results, it is evident that universum twin support vector machine (UTSVM) is better in comparison to other support vector machine algorithms for EEG signal classification. Among the different feature extraction techniques, ICA shows the best results using our proposed approach with 99 % accuracy. The proposed work also gives a comparison of the different SVM based algorithms for the classification of EEG signals. It is evident from the experimental results that other variants of SVM such as TWSVM and UTSVM give good generalization and computational performance and are applicable for the classification of EEG signals. The universum based SVM approach needs to be applied to other diseases which are diagnosed using EEG signals with the proper selection of universum. In future, our universum based approach of EEG classification can be improved in terms of computational time. Our proposed universum based approach can be extended to multiclass classification of EEG signals using EEG datasets generated with different feature extraction techniques.