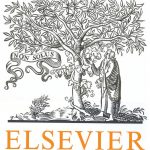
ترجمه مقاله نقش ضروری ارتباطات 6G با چشم انداز صنعت 4.0
- مبلغ: ۸۶,۰۰۰ تومان
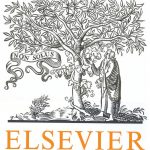
ترجمه مقاله پایداری توسعه شهری، تعدیل ساختار صنعتی و کارایی کاربری زمین
- مبلغ: ۹۱,۰۰۰ تومان
abstract
In contrast to classical experiment design methods, often based on alphabetic criteria, economic optimal experiment design assumes that our ultimate goal is to solve an optimization or optimal control problem. As the system parameters of physical models are in practice always estimated from measurements, they cannot be assumed to be exact. Thus, if we solve the model based optimization problem using the estimated, non-exact parameters, an inevitable loss of optimality is faced. The aim of economic optimal experiment design is precisely to plan an experiment in such a way that the expected loss of optimality in the optimization is minimized. This paper analyzes the question how to design economic experiments under the assumption that we have more than one candidate objective function. Here, we want to take measurements and estimate the parameters before we actually decide which objective we want to minimize.
6. Conclusions
In this paper, we have introduced a novel class of economic objectives for optimal experiment design under the assumption that the estimated parameters shall be used in possibly more than one application. A key contribution of this paper is presented in Theorem 3.1, where we have proven that min-max multi-purpose economic optimal experiment design problems can be reformulated as standard nonlinear programming problems, which makes these problems tractable for solving with standard nonlinear programming problem solvers. We have illustrated the practical applicability oftheproposedformulation withtwodifferent case studies, i.e., an illustrative model describing the growth of micro algae and a model describing the induced foreign protein production by recombinant bacteria. We observed that the multi-purpose approach leads in the first case study to a reduction ofthe worst case expected optimality gap by a factor compared with the A-optimal design while for the second case study a reduction of 18% is observed. In addition, these predictions are validated by Monte Carlo simulations which confirm the predicted expected optimality losses.