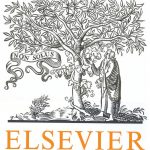
ترجمه مقاله نقش ضروری ارتباطات 6G با چشم انداز صنعت 4.0
- مبلغ: ۸۶,۰۰۰ تومان
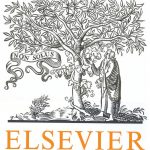
ترجمه مقاله پایداری توسعه شهری، تعدیل ساختار صنعتی و کارایی کاربری زمین
- مبلغ: ۹۱,۰۰۰ تومان
Abstract
In video surveillance, face recognition (FR) systems are employed to detect individuals of interest appearing over a distributed network of cameras. The performance of still-tovideo FR systems can decline significantly because faces captured in unconstrained operational domain (OD) over multiple video cameras have a different underlying data distribution compared to faces captured under controlled conditions in the enrollment domain (ED) with a still camera. This is particularly true when individuals are enrolled to the system using a single reference still. To improve the robustness of these systems, it is possible to augment the reference set by generating synthetic faces based on the original still. However, without knowledge of the OD, many synthetic images must be generated to account for all possible capture conditions. FR systems may, therefore, require complex implementations and yield lower accuracy when training on many less relevant images. This paper introduces an algorithm for domain-specific face synthesis (DSFS) that exploits the representative intra-class variation information available from the OD. Prior to operation (during camera calibration), a compact set of faces from unknown persons appearing in the OD is selected through affinity propagation clustering in the captured condition space (defined by pose and illumination estimation). The domainspecific variations of these face images are then projected onto the reference still of each individual by integrating an imagebased face relighting technique inside the 3D reconstruction framework. A compact set of synthetic faces is generated that resemble individuals of interest under the capture conditions relevant to the OD. In a particular implementation based on sparse representation classification, the synthetic faces generated with the DSFS are employed to form a cross-domain dictionary that accounts for structured sparsity where the dictionary blocks combine the original and synthetic faces of each individual. Experimental results obtained with videos from the Chokepoint and COX-S2V datasets reveal that augmenting the reference gallery set of still-to-video FR systems using the proposed DSFS approach can provide a significantly higher level of accuracy compared to state-of-the-art approaches, with only a moderate increase in its computational complexity.
CONCLUSIONS
This paper proposes a domain-specific face synthesizing (DSFS) technique to improve the performance of still-to-video FR systems when surveillance videos are captured under various uncontrolled conditions, and individuals are recognized based on a single facial image. The proposed approach takes advantage of operational domain information from the generic set that can effectively represent probe ROIs. A compact set of synthetic faces is generated that resemble individuals of interest under capture conditions relevant to the operational domain. For proof-of-concept validation, an augmented dictionary with a block structure is designed based on DSFS, and face classification is performed within a SRC framework. Our experiments on the Chokepoint and COX-S2V datasets show that augmenting the reference discretionary of still-tovideo FR systems using the proposed DSFS approach can provide a higher level of accuracy compared to state-of-the-art approaches, with only a moderate increase in its computational complexity. The results indicated that face synthesis alone (without recovering the OD information) cannot effectively resolve the challenges of the SSPP and visual domain shift problems. With DSFS, generic learning and face synthesis operate complementarity. The proposed DSFS technique could be improved to generate synthetic faces with expression variations for a robust FR. In addition, to improve performance, the representative synthetic ROIs generated using DSFS could be applied to generate local camera-specific ROIs. DSFS is general in that synthetic ROIs could be applied to train or finetune a multitude of face recognition systems like deep CNNs, with information that robust models to specific operational domains.